Understanding Beagle Obesity Causes and Consequences
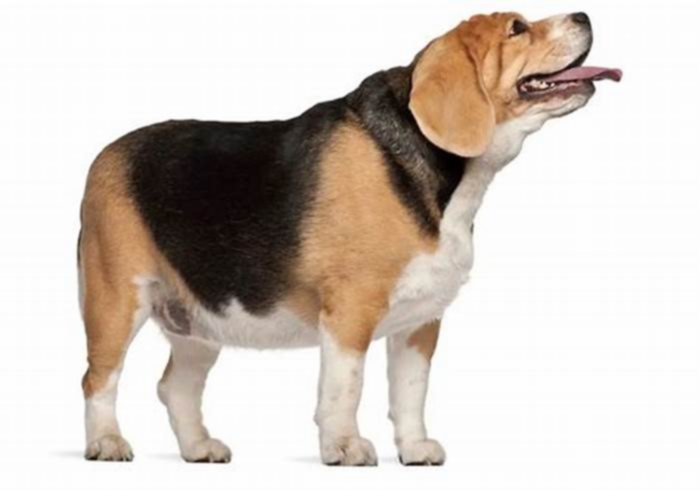
Obesity: causes, consequences, treatments, and challenges
Obesity has become a global epidemic and is one of todays most public health problems worldwide. Obesity poses a major risk for a variety of serious diseases including diabetes mellitus, non-alcoholic liver disease (NAFLD), cardiovascular disease, hypertension and stroke, and certain forms of cancer (Bluher, 2019).
Obesity is mainly caused by imbalanced energy intake and expenditure due to a sedentary lifestyle coupled with overnutrition. Excess nutrients are stored in adipose tissue (AT) in the form of triglycerides, which will be utilized as nutrients by other tissues through lipolysis under nutrient deficit conditions. There are two major types of AT, white AT (WAT) and brown AT, the latter is a specialized form of fat depot that participates in non-shivering thermogenesis through lipid oxidation-mediated heat generation. While WAT has been historically considered merely an energy reservoir, this fat depot is now well known to function as an endocrine organ that produces and secretes various hormones, cytokines, and metabolites (termed as adipokines) to control systemic energy balance. Studies over the past decade also show that WAT, especially subcutaneous WAT, could undergo beiging remodeling in response to environmental or hormonal perturbation. In the first paper of this special issue, Cheong and Xu (2021) systematically review the recent progress on the factors, pathways, and mechanisms that regulate the intercellular and inter-organ crosstalks in the beiging of WAT. A critical but still not fully addressed issue in the adipose research field is the origin of the beige cells. Although beige adipocytes are known to have distinct cellular origins from brown and while adipocytes, it remains unclear on whether the cells are from pre-existing mature white adipocytes through a transdifferentiation process or from de novo differentiation of precursor cells. AT is a heterogeneous tissue composed of not only adipocytes but also nonadipocyte cell populations, including fibroblasts, as well as endothelial, blood, stromal, and adipocyte precursor cells (Ruan, 2020). The authors examined evidence to show that heterogeneity contributes to different browning capacities among fat depots and even within the same depot. The local microenvironment in WAT, which is dynamically and coordinately controlled by inputs from the heterogeneous cell types, plays a critical role in the beige adipogenesis process. The authors also examined key regulators of the AT microenvironment, including vascularization, the sympathetic nerve system, immune cells, peptide hormones, exosomes, and gut microbiota-derived metabolites. Given that increasing beige fat function enhances energy expenditure and consequently reduces body weight gain, identification and characterization of novel regulators and understanding their mechanisms of action in the beiging process has a therapeutic potential to combat obesity and its associated diseases. However, as noticed by the authors, most of the current pre-clinical research on beiging are done in rodent models, which may not represent the exact phenomenon in humans (Cheong and Xu, 2021). Thus, further investigations will be needed to translate the findings from bench to clinic.
While both socialenvironmental factors and genetic preposition have been recognized to play important roles in obesity epidemic, Gao et al. (2021) present evidence showing that epigenetic changes may be a key factor to explain interindividual differences in obesity. The authors examined data on the function of DNA methylation in regulating the expression of key genes involved in metabolism. They also summarize the roles of histone modifications as well as various RNAs such as microRNAs, long noncoding RNAs, and circular RNAs in regulating metabolic gene expression in metabolic organs in response to environmental cues. Lastly, the authors discuss the effect of lifestyle modification and therapeutic agents on epigenetic regulation of energy homeostasis. Understanding the mechanisms by which lifestyles such as diet and exercise modulate the expression and function of epigenetic factors in metabolism should be essential for developing novel strategies for the prevention and treatment of obesity and its associated metabolic diseases.
A major consequence of obesity is type 2 diabetes, a chronic disease that occurs when body cannot use and produce insulin effectively. Diabetes profoundly and adversely affects the vasculature, leading to various cardiovascular-related diseases such as atherosclerosis, arteriosclerotic, and microvascular diseases, which have been recognized as the most common causes of death in people with diabetes (Cho et al., 2018). Love et al. (2021) systematically review the roles and regulation of endothelial insulin resistance in diabetes complications, focusing mainly on vascular dysfunction. The authors review the vasoprotective functions and the mechanisms of action of endothelial insulin and insulin-like growth factor 1 signaling pathways. They also examined the contribution and impart of endothelial insulin resistance to diabetes complications from both biochemical and physiological perspectives and evaluated the beneficial roles of many of the medications currently used for T2D treatment in vascular management, including metformin, thiazolidinediones, glucagon-like receptor agonists, dipeptidyl peptidase-4 inhibitors, sodium-glucose cotransporter inhibitors, as well as exercise. The authors present evidence to suggest that sex differences and racial/ethnic disparities contribute significantly to vascular dysfunction in the setting of diabetes. Lastly, the authors raise a number of very important questions with regard to the role and connection of endothelial insulin resistance to metabolic dysfunction in other major metabolic organs/tissues and suggest several insightful directions in this area for future investigation.
Following on from the theme of obesity-induced metabolic dysfunction, Xia et al. (2021) review the latest progresses on the role of membrane-type I matrix metalloproteinase (MT1-MMP), a zinc-dependent endopeptidase that proteolytically cleaves extracellular matrix components and non-matrix proteins, in lipid metabolism. The authors examined data on the transcriptional and post-translational modification regulation of MT1-MMP gene expression and function. They also present evidence showing that the functions of MT1-MMP in lipid metabolism are cell specific as it may either promote or suppress inflammation and atherosclerosis depending on its presence in distinct cells. MT1-MMP appears to exert a complex role in obesity for that the molecule delays the progression of early obesity but exacerbates obesity at the advanced stage. Because inhibition of MT1-MMP can potentially lower the circulating low-density lipoprotein cholesterol levels and reduce the risk of cancer metastasis and atherosclerosis, the protein has been viewed as a very promising therapeutic target. However, challenges remain in developing MT1-MMP-based therapies due to the tissue-specific roles of MT1-MMP and the lack of specific inhibitors for this molecule. Further investigations are needed to address these questions and to develop MT1-MMP-based therapeutic interventions.
Lastly, Huang et al. (2021) present new findings on a critical role of puromycin-sensitive aminopeptidase (PSA), an integral non-transmembrane enzyme that catalyzes the cleavage of amino acids near the N-terminus of polypeptides, in NAFLD. NAFLD, ranging from simple nonalcoholic fatty liver to the more aggressive subtype nonalcoholic steatohepatitis, has now become the leading chronic liver disease worldwide (Loomba et al., 2021). At present, no effective drugs are available for NAFLD management in the clinic mainly due to the lack of a complete understanding of the mechanisms underlying the disease progress, reinforcing the urgent need to identify and validate novel targets and to elucidate their mechanisms of action in NAFLD development and pathogenesis. Huang et al. (2021) found that PSA expression levels were greatly reduced in the livers of obese mouse models and that the decreased PSA expression correlated with the progression of NAFLD in humans. They also found that PSA levels were negatively correlated with triglyceride accumulation in cultured hepatocytes and in the liver of ob/ob mice. Moreover, PSA suppresses steatosis by promoting lipogenesis and attenuating fatty acid -oxidation in hepatocytes and protects oxidative stress and lipid overload in the liver by activating the nuclear factor erythroid 2-related factor 2, the master regulator of antioxidant response. These studies identify PSA as a pivotal regulator of hepatic lipid metabolism and suggest that PSA may be a potential biomarker and therapeutic target for treating NAFLD.
In summary, papers in this issue review our current knowledge on the causes, consequences, and interventions of obesity and its associated diseases such as type 2 diabetes, NAFLD, and cardiovascular disease (Cheong and Xu, 2021; Gao et al., 2021; Love et al., 2021). Potential targets for the treatment of dyslipidemia and NAFLD are also discussed, as exemplified by MT1-MMP and PSA (Huang et al., 2021; Xia et al., 2021). It is noted that despite enormous effect, few pharmacological interventions are currently available in the clinic to effectively treat obesity. In addition, while enhancing energy expenditure by browning/beiging of WAT has been demonstrated as a promising alternative approach to alleviate obesity in rodent models, it remains to be determined on whether such WAT reprogramming is effective in combating obesity in humans (Cheong and Xu, 2021). Better understanding the mechanisms by which obesity induces various medical consequences and identification and characterization of novel anti-obesity secreted factors/soluble molecules would be helpful for developing effective therapeutic treatments for obesity and its associated medical complications.
References
Bluher
M.
(2019
).
Obesity: global epidemiology and pathogenesis
.
Nat. Rev. Endocrinol
.
15
,
288
298
.
Cheong
L.Y.
,Xu
A.
(2021
).
Intercellular and inter-organ crosstalk in browning of white adipose tissue: molecular mechanism and therapeutic complications
.
J. Mol. Cell Biol
.
13
,
466
479
.
Cho
N.H.
,Shaw
J.E.
,Karuranga
S.
, et al. (2018
).
IDF Diabetes Atlas: global estimates of diabetes prevalence for 2017 and projections for 2045
.
Diabetes Res. Clin. Pract
.
138
,
271
281
.
Gao
W.
,Liu
J.-L.
,Lu
X.
, et al. (2021
).
Epigenetic regulation of energy metabolism in obesity
.
J. Mol. Cell Biol
.
13
,
480
499
.
Huang
B.
,Xiong
X.
,Zhang
L.
, et al. (2021
).
PSA controls hepatic lipid metabolism by regulating the NRF2 signaling pathway
.
J. Mol. Cell Biol
.
13
,
527
539
.
Loomba
R.
,Friedman
S.L.
,Shulman
G.I.
(2021
).
Mechanisms and disease consequences of nonalcoholic fatty liver disease
.
Cell
184
,
2537
2564
.
Love
K.M.
,Barrett
E.J.
,Malin
S.K.
, et al. (2021
).
Diabetes pathogenesis and management: the endothelium comes of age
.
J. Mol. Cell Biol
.
13
,
500
512
.
Ruan
H.-B.
(2020
).
Developmental and functional heterogeneity of thermogenic adipose tissue
.
J. Mol. Cell Biol
.
12
,
775
784
.
Xia
X.-D.
,Alabi
A.
,Wang
M.
, et al. (2021
).
Membrane-type I matrix metalloproteinase (MT1-MMP), lipid metabolism, and therapeutic implications
.
J. Mol. Cell Biol
.
13
,
513
526
.
Author notes
The Author(s) (2021). Published by Oxford University Press on behalf of Journal of Molecular Cell Biology, CEMCS, CAS.
This is an Open Access article distributed under the terms of the Creative Commons Attribution-NonCommercial License (
https://creativecommons.org/licenses/by-nc/4.0/), which permits non-commercial re-use, distribution, and reproduction in any medium, provided the original work is properly cited. For commercial re-use, please contact [email protected]
A systematic literature review on obesity: Understanding the causes & consequences of obesity and reviewing various machine learning approaches used to predict obesity
Computers in Biology and Medicine 136 (2021) 104754Contents lists available at ScienceDirectComputers in Biology and Medicinejournal homepage: www.elsevier.com/locate/compbiomedA systematic literature review on obesity: Understanding the causes &consequences of obesity and reviewing various machine learningapproaches used to predict obesityMahmood Safaei a, Elankovan A. Sundararajan a, *, Maha Driss b, c, Wadii Boulila b, c,Azrulhizam Shapii daCentre for Software Technology and Management, Faculty of Information Science and Technology, Universiti Kebangsaan Malaysia (UKM), Bangi, 43600, Selangor,MalaysiabRIADI Laboratory, University of Manouba, Manouba, TunisiacCollege of Computer Science and Engineering, Taibah University, Medina, Saudi ArabiadCenter for Artificial Intelligence Technology, Faculty of Information Science and Technology, Universiti Kebangsaan Malaysia (UKM), Bangi, 43600, Selangor,MalaysiaA R T I C L E I N F OA B S T R A C TKeywords:ObesityOverweightMachine learningRisk factorsDiseasesObesity is considered a principal public health concern and ranked as the fifth foremost reason for death globally.Overweight and obesity are one of the main lifestyle illnesses that leads to further health concerns and contributes to numerous chronic diseases, including cancers, diabetes, metabolic syndrome, and cardiovasculardiseases. The World Health Organization also predicted that 30% of death in the world will be initiated withlifestyle diseases in 2030 and can be stopped through the suitable identification and addressing of associated riskfactors and behavioral involvement policies. Thus, detecting and diagnosing obesity as early as possible iscrucial. Therefore, the machine learning approach is a promising solution to early predictions of obesity and therisk of overweight because it can offer quick, immediate, and accurate identification of risk factors and conditionlikelihoods. The present study conducted a systematic literature review to examine obesity research and machinelearning techniques for the prevention and treatment of obesity from 2010 to 2020. Accordingly, 93 papers areidentified from the review articles as primary studies from an initial pool of over 700 papers addressing obesity.Consequently, this study initially recognized the significant potential factors that influence and cause adultobesity. Next, the main diseases and health consequences of obesity and overweight are investigated. Ultimately,this study recognized the machine learning methods that can be used for the prediction of obesity. Finally, thisstudy seeks to support decision-makers looking to understand the impact of obesity on health in the generalpopulation and identify outcomes that can be used to guide health authorities and public health to furthermitigate threats and effectively guide obese people globally.1. IntroductionObesity and its attendant conditions have become major healthproblems worldwide, and obesity is currently ranked as the fifth mostcommon leading cause of death globally. The World Health Organization (WHO) defines obesity as an abnormal or excessive fat accumulation that may impair health, further clarifying that the fundamentalcause of obesity and overweight is an energy imbalance between calories consumed and calories expended [1,2]. The unit of Body MassIndex (BMI), which is measured by calculating [(weight in kg)/(heightin m2)], is a simple index intended to classify adults into one of threecategories: underweight, overweight, or obese. Though initiallydeveloped in the 1830s by a Belgian mathematician and sociologist, BMIis still widely used as a measurement of obesity and obesity rates. Forinstance, the WHO [3], often classifies adult obesity using certain BMIcutoffs (Table 1). This WHO classification is beneficial in distinguishingindividuals who may be at increased risk of morbidity and mortality dueto obesity. Over the last two decades, the rates of obesity (calculated asadults having BMI over 30 kg/m2) have rapidly increased across thedeveloping world. Researchers have estimated that numbers reached* Corresponding author.E-mail address: [email protected] (E.A. Sundararajan).https://doi.org/10.1016/j.compbiomed.2021.104754Received 26 April 2021; Received in revised form 5 August 2021; Accepted 5 August 2021Available online 16 August 20210010-4825/ 2021 The Author(s). Published by Elsevier Ltd. This is an open access article under the CC BY license (http://creativecommons.org/licenses/by/4.0/).M. Safaei et al.Computers in Biology and Medicine 136 (2021) 104754 To identify the ML techniques currently used in the automatic prediction and/or identification of adult obesity.Table 1BMI classification of adult weights based on WHO schema (BMI = weight in kg/height in meters2).ClassificationB2.5UnderweightNormal weightOverweightObeseObese IObese IIObese IIIBMI (kg/m2 )<18.518.524.925.029.93030.034.935.039.940The remainder of this paper is organized as follows. Section 2 discusses related works from across the current scientific literature. Section3 describes the proposed methodology for an SLR process related toobesity, and the overall process of the project. Section 4 presents theresults and discussions, while Section 5 discusses future directions andapplications of this work. Table 2 displays the list of acronyms used inthis study.Risk of co-morbiditiesLow (but risk of other clinical problemsincreased)AverageMildly increasedModerateSevereVery severe2. Related research worksObesity is commonly recognized as a critical public health issue andhas drawn significant interest across the health sciences. In addition tooriginal research using traditional scientific methods, studies in this areahave discussed prevention, treatment, and quality of life for those livingwith obesity, often through SLRs and novel methods, such as ML. Thissection summarizes several related studies in preparation for comparisons with the current work and offers an overview of the current literature addressing obesity from several perspectives.Simmonds et al. [14] conducted a systematic review later combinedwith meta-analysis to examine whether BMI and similar measures usedto calculate childhood obesity could also predict adult obesity. Theirreview supported the conclusion that teenage obesity is a notable publichealth crisis because, it often continues into adulthood. Accordingly,acting to reduce teen obesity can also reduce adult obesity. Early actionis one of the most suitable approaches because once children havebecome overweight, this trend often persists through their adolescenceand adulthood.More recently, de Siqueira et al. [15] presented a survey that evaluated potential relationships between obesity and COVID-19, as tracedthrough increased hospitalization rates, poor diagnosis and recoveryoutcomes, and high death rates. This survey identified and validatedassociations between high BMI (that is, significantly higher than 30kg/msup2) and poor COVID-19 outcomes. The researchers also discerned a high severity of clinical COVID-19 and a considerable641 million obese adults in 2014 compared to only 105 million in 1975[4], thus showing an alarming increase [5]. Multiple studies havedemonstrated that obesity is not a simple problem but a complex healthissue stemming from a combination of individual factors (genetics,learned behaviors) and substantial causes (unhealthy societal or culturaleating habits, food deserts) [6,7]. Most researchers also agree thatobesity is an acquired disease that, heavily depends on lifestyle factors(i.e., personal choices), such as low rates of physical activity and chronicovereating, despite its genetic and epigenetic influences. Researchershave also noted that various forms of obesity, including abdominalobesity, are related to increased risk of several chronic conditions anddiseases, which include asthma, cancer, diabetes, hypercholesterolemia,and, cardiovascular diseases [8,9]. Thus, while obesity is undoubtedly acondition, it also exacerbates pre-existing conditions and instigates newones [10]. More specifically, Bischoff et al. [11] maintained that obesitycan affect nearly every organ system, from the cardiovascular (CV)system to the endocrine system, central nervous system, and thegastrointestinal (GI) system. In addition, obesity is associated with thegrowing prevalence of several CV conditions, from hypertension andcoronary heart disease (CHD) to atrial fibrillation (AF) and even totalheart failure [12].Some genetic and lifestyle factors affect an individuals likelihood ofadult obesity; thus, the significant clusters of obesity observed in specificgeographical regions and contexts also signal the impact of socioeconomic and environmental factors in obesogenic environments [13].Understanding the causes and determinants of obesity is a critical steptoward creating effective policy and developing workable preventionprograms due to the aforementioned additional complications. Effortswill not succeed without detailed, science-based understandings of therisk factors for obesity and the numerous links among these factors.Although several studies have concentrated on overweight andobesity, systematic literature reviews (SLRs) and similar overviews thatoutline the potential parameters influencing and causing adult obesityare still needed. Similarly, SLRs are necessary to identify overlaps between studies of obesity and research methods such as machine learning(ML). Limited studies have investigated how ML techniques can predictadult obesity. however, groundbreaking work in any effective, systematic manner has not been presented to date. Therefore, this SLR systematically investigates the causes of adult obesity and the current andemerging research in this investigation. As briefly proposed above, thisSLR is intended to support practitioners and decision-makers by helpingthem take useful information from the existing literature. Therefore, thisstudy will support mergers among cutting-edge research, medicalknowledge, and policy makers to propose new evidence-based approaches and solutions regarding adult obesity. The major contributionsof this SLR include the following:Table 2List of abbreviations and acronyms.AcronymDescriptionAcronymDescriptionWHOMLSLRWorld Health OrganizationMachine LearningSystematic Literature ReviewLRNBANNsBMIBody Mass IndexRNNCVGICardiovascularGastrointestinalGBGFACHDAFQACoronary Heart DiseaseAtrial FibrillationQuality AssessmentADPDBPHRQAIResearch QuestionsArtificial IntelligencePCaRAMLFFNNMulti-Layer Perceptron FeedForward Artificial NeuralNetworksMillennium Cohort StudySLELogistic RegressionNaive BayesArtificial NeuralNetworksRecurrent NeuralNetworkGradient BoostingGroup FactorAnalysisAlzheimers DiseaseParkinsons DiseaseBenign ProstateHyperplasiaProstate CancerRheumatoidArthritisSystemic LupusErythematosusSupport Vector MachineRegression ModelDecision TreeRanked Guided Iterative FeatureEliminationDeep Convolutional NeuralNetworksK-Nearest NeighborsMSMCSSVM To identify existing potential parameters that influence and causeadult obesity. To investigate major diseases, conditions, and other negative healtheffects related to adult obesity and overweightness.DTRGIFEDCNNKNN2IBDT1DTAIHTRFInflammatory BowelDiseaseMultiple SclerosisType-1 DiabetesThyroidAutoimmunityHashimotoThyroiditisRandom ForestM. Safaei et al.Computers in Biology and Medicine 136 (2021) 104754prevalence of ICU and general hospitalizations in patients with highBMI. Overall, researchers concluded that obesity was an adverse determinant for COVID-19. Specifically, high BMI led to worse outcomes.Ananthakumar et al. [16] also presented a survey evaluating patientresponses to consultations regarding extra weight. Their research alsoevaluated the role of physician weight in framing the responses and howthe observed significance of extra body weight to specific health situations shaped those responses. Moreover, this survey developed a theoretical understanding of common motivations behind such reactions.This review concluded that patients could be anticipated to answerhonestly to clinicians inquiring into current attempts to lose weight.These findings indicated that, weight loss investigations were also successful when they involved a trusted clinician who took time toenumerate the advantages of weight loss in a non-judgmental way.Felso et al. [17] also presented an SLR studying associations betweenduration of sleep and childhood obesity. They also examined the physiological and pathophysiological mechanisms that might underlie thisconnection. Their work found that sleep duration affects weight increasein children, despite the unknown precise mechanisms. Their findingsalso confirmed that additional factors, such as a sedentary lifestyle,unhealthy diet, and insulin resistance, can all predispose children topoor sleep, and by extension, to unhealthy weight gains as well. Felsoet al. [17] also reported that the role of other supposed mediators(ghrelin, screen time, and leptin levels) remains uncertain. Overall, mostof the literature on obesity focus on exploring the potential parametersthat cause, impact, and/or worsen obesity in adults considering therepresentative samples. ML techniques have also been used in severalhealth applications, including disease recognition, over the last severalyears. However, only limited research has focused on associations between ML approaches and obesity. Moreover, understanding the potential association of obesity and its chronic diseases with severeoutcomes is vital but still often neglected in previous studies.The present SLR aims to address these gaps through the following:Fig. 1. Research process.research protocol and its processes are outlined in Fig. 2.3.2. Identifying research questionsThe following research questions (RQ) were formulated for thisparticular study.RQ1 What are the factors that potentially influence and/or cause adultobesity?RQ2 What are the most important applied ML techniques currentlyused for predicting obesity issues?RQ3 What are the major medical conditions and diseases associatedwith obesity, based on the current literature?3.3. Search strategy Locating the most significant works related to obesity, their causes,and their risk factors. Identifying the ML techniques used most often and productively topredict obesity. Surveying associations between obesity and other risks, conditions,and diseases.In the current work, a backward and forward search approach wasfirst applied to determine the reference of the chosen studies. TheGoogle Scholar search engine was utilized to go forward and obtainthe articles mentioned within selected initial reviews [22]. This searchstrategy is significant due to its data extraction capability from particular articles, and aiding researchers in locating as many related papersas possible.Thus, select databases and libraries were utilized to locate the mostrelevant articles to the formulated research questions. The selectedkeywords are as follows:3. Research methodsA systematic literature review (SLR) describes a coordinated, systembased means of devising relevant research questions, defining importantkeywords, and finally collating collected research in an accessible, systematic way [1820]. An SLR will analyze a particular problem broadlyand offer alternative methods for investigating the issue comprehensively, as further demonstrated below. The next sub-sections demonstrate the research methodology and the SLR protocol used to conductthis study. Obesity OR Overweight AND Factors OR Parameters; Obesity OR Overweight AND Machine learning techniques; Obesity OR Overweight AND Disorders OR Diseases.The following online databases were included in the search strategy,limiting results to a range of 10 years (from September 2010 toSeptember 2020):3.1. Review protocolThis study follows the standards introduced by Kitchenham andCharters [18], and also uses Jula [21] who maintained that the SLRmethod comprises the following three phases: (1) planning the SLR, (2)conducting the research for the SLR, and (3) reporting the outcomes ofthe SLR. Each of the three main steps also includes the followingparticular tasks: proposing the driving research questions, developingthe review protocol, describing the inclusion and exclusion criteria,selecting a viable search strategy, developing the resulting study process, and planning for quality assessment (QA), data extraction, andsynthesis of findings. Subsequent sections of this paper describe theproposed approach to these steps comprehensively. Meanwhile, Fig. 1displays the steps of conducting an SLR, while the overall recommendedScience DirectSpringer LinkIEEE ExplorerTaylor and Francis OnlineACM Digital LibraryMDPINCBIA manual search was then conducted after the initial automatedsearch. Watson and Webster [23] maintained that a forward andbackward search approach can be used to trace locations for the references cited in primary studies. This manual step was adopted3M. Safaei et al.Computers in Biology and Medicine 136 (2021) 104754Fig. 2. Review protocol.following the initial search to ensure the systematicity, comprehensiveness, and completeness of this SLR. Mendeley, an industry-standardreference management tool, was then employed to arrange and classifythe selected articles, including the retainment of all research results andthe elimination of duplicates.Table 3Inclusion and exclusion criteria.3.4. Inclusion and exclusion criteriaCriteriaPrincipleInclusionStudies published within a particular time period (Jan. 2010Oct. 2020)Studies written in EnglishStudies that were completeStudies related to the defined research questionsDuplicated studiesStudies in languages other than EnglishStudies whose work was irrelevant to the research questions of thecurrent studyExclusionNext, inclusion (characteristics that qualify subjects for inclusion)and exclusion (characteristics that disqualify subjects from inclusion)criteria were identified to ensure the relationship of the located articlesto the subject of this research work.First, abstracts were read closely to decide whether they addressedsubjects, approaches, or other information that fit the scope of the current SLR. Second, each article was reviewed considering specific inclusion and exclusion criteria to determine whether or not to retain them.Only articles published in English, on the subject of obesity, within aspecific period (January 2010October 2020) were ultimately selectedfor this particular review. Table 3 summarizes the specific inclusion andexclusion criteria utilized in this research.3.5. Study selection processThe selection process mainly aims to identify relevant articles. Atotal of 738 articles were initially obtained from automatic searches4M. Safaei et al.Computers in Biology and Medicine 136 (2021) 104754based on the keywords defined above. The results were narrowed to only296 articles after eliminating repeated articles using the referencemanagement tool Mendeley. Inclusion and exclusion criteria were thenutilized to review the abstract and conclusion of each study, whichremoved 167 articles from consideration. Any irrelevant articles to theresearch questions were also removed in accordance with guidelines ofKitchenham and Charters [24].Exclusion criteria were then applied to the full articles, using full-textscanning to determine whether articles with abstracts and conclusionsthat initially meet the inclusion criteria were still fit. Manual searches atthe reference page level of individual articles also used to identify anyarticles that might have been missed with initial searches. This manualsearch further obtained 8 studies, while the scans eliminated over 30. Atotal of 137 primary studies were considered after the completion of theaforementioned step. The final step was to implement quality assessment (QA) measures, which eliminated 44 additional papers, retaining atotal of 110 articles as the primary studies of the SLR. (For reference, thebibliographic information of all included works is listed in Table in theAppendix).Table 4Data extracted from the primary studies included.ExtracteddataDescriptionStudy IDPaper TitleYearResearchtopicCountryMethodCausingfactorsDiseaseUnique number assigned to each study protocolTitle of each study, as presented in the articleYear of publication for each articleDescription of what each study addressedCountries addressed by each studyML technique(s) used in each studyCauses and factors that often lead to obesity, as mentioned and/oraddressed in each studyMajor disease related with obesity and overweightness, asmentioned and/or addressed in each studybetween January 2010 and October 2020. The distribution of articlespublished within this period is demonstrated in Fig. 3, which shows thattheir prevalence has steadily risen since 2011. Other notable milestonesinclude the most significant number of papers in a single year, whichwas 2017 with 19 published articles, followed by 2020 with 15 published studies. This trend reveals the increase in investigations onobesity over time, particularly in the last decade.In a follow-up to this first temporal view, Google searches for theterms Obesity and then Machine learning, were also ran to determine their use during a comparable time period (from 1 January 2010through 18 November 2020). This approach was conducted to discernwhether Google searches would also reflect the observed increase withthe publishing rate discussed above. A match herein would furtherhighlight the current interest in and importance of this combinedresearch domain.Therefore, the Google Trends tool (Trend, 2020) was used to targetthe two keywords: Obesity and Machine learning. As demonstratedin Fig. 4, the increasing prevalence of search queries in this area suggeststhat most users were more interested in Machine learning thanObesity.3.6. Quality assessmentQA criteria help researchers answer pre-determined research questions and ensure that the purposes of the study are met [25]. Thefollowing criteria were used for the QA process:QA1 Are the topics presented in each article associated with the reviewsubject?QA2 Is the methodology of the study described precisely in eacharticle?QA3 Is the data gathering process of the researchers explained in eacharticle?QA4 Are the data analysis method of the researchers illustrated in eacharticle?The four QA criteria were then used to further assess the quality,applicability, credibility, and suitability of the 110 articles selected inprevious steps.The four-question QA schema was suggested by Nidhra et al. [26],who used such questions to assign each collected article one of threelevels of quality (high, medium, low). Herein, the level of qualityassigned to each article depends on the sum of the calculated consequences for all QA criteria. Studies that meet all criteria will be awardeda score of 2, those that moderately satisfy criteria will be awarded ascore of 1, and, studies that do not satisfy any criteria will be awarded ascore of 0. Overall, studies that received a score of 5 or higher on thisscale were of high quality, while those with a score of 4 were ratedaverage. Meanwhile, studies that received scores lower than 4 were oflow quality and eliminated from consideration in this SLR. A total of 44further articles were excluded.4. Research questions resultsRQ1: What are the potential factors that influence and cause adultobesity?High BMI in adults (specifically, (25 kg/m2) has been shown tocomplicate, or even lead to, high occurrences of cardiovascular diseases,conditions, and issues, as well as rising mortality rates [27]. Thus, understanding the common causes of obesity and excessive weight gain iscrucial because this will enable the development of approaches and evenpolicies that could help curb this global epidemic [5]. Current researchhas already identified or verified certain unhealthy habits, including3.7. Extraction and synthesis of dataData extraction was performed to obtain relevant information fromeach article to be included in the SLR. The reference management toolMendeley and Microsoft Excel spreadsheets were utilized to collate andcompare important information.This process began with the creation of a form to manage theextracted data, in the form of columns for several relevant items fromeach of the 93 studies to be included. The created columns were allocated to the extracted data, which included ID, year of publication, andML method(s) or approach(es) of each study. Table 4 presents the fulldata extraction form used for the 93 studies.3.7.1. Temporal view of publicationAs specified in Section 2.4, this study focused on articles publishedFig. 3. Temporal view of primary studies.5M. Safaei et al.Computers in Biology and Medicine 136 (2021) 104754Table 5Potential factors that influence and/or cause obesity.Author (s)Factor(s)Cheng [38]Maternal smokingNeurological functioningPhysical exercisePersonality and/or intelligencePhysical inactivityLacking and/or imbalanced dietSocio-economic status (past or present)Diabetes and/or glucose intoleranceGenderHigh maternal BMIMaternal level of educationHigh birth weightMetabolic syndromeHypertensionDyslipidemiaEducation levelEating meals at regular timesLength of sleep timeFrequency of physical exerciseIntensity of stress and/or depressionAnnual household incomeSubjective satisfaction with daily lifeSubjective satisfaction with personal healthLifestyle factorsConsumption of fruits and vegetablesExcess drinking or alcohol consumption, past or presentLacking or insufficient physical activityHealth factorsPsychological distressSocio-demographic factorsAgeSexMarital statusEducationLabor force statusEthnicityRemotenessPhysical functioningChoukem et al. [31]Fig. 4. Number of Google results for the terms obesity and machine learning.Ishida et al. [29]excess drinking, smoking, insufficient exercise, and overeating, as directcauses of obesity and other chronic illnesses [28].However, obesity also has multiple impacting factors. Table 5 depictssome of the influential factors that determine adult overweightness orobesity, as reported by previous researchers. For instance, Ishida et al.[29] conducted a study showing the following: (1) those who are not ingood health tend to be lean; (2) middle-aged people, those who eatirregularly, and those with low standards of living are often overweight;and finally, (3) the middle-aged, those suffering from stress anddepression, those with low life satisfaction, and those who bring in lowannual household incomes are often obese.Several studies have also indicated infrequency or lack of exerciseand individual education levels as determining factors of obesity[2934]. Further study by Kadouh and Acosta [5,35] have also shownthat obesity may be similar to a heterogeneous chronic condition, inwhich numerous factors interact, producing an energy imbalance thatleads to increased body weight. Thus, biological, environmental, andbehavioral factors are all determinants of obesity. Accordingly, differences in the prevalence of obesity among different population groupscould be influenced by various behavioral and environmental factors,predominantly increasing caloric consumption and reduced physicalactivity [36,37]. Fig. 5 also illustrates factors that often lead to obesity.Another study conducted by Cheng et al. [38] revealed that significant predictors of adult obesity, particularly around the age of 55(women and men), include maternal smoking during pregnancy, childhood neurological functions, educational qualifications, trait conscientiousness, and physical exercise. An earlier study by Cheng and Furnham[30] also identified many of the same traits and maintained that eachwas significantly, but also independently, associated with chances ofadult obesity.Keramat et al. [49]Pinto Pereira et al.[50]Sun et al. [37]Sun et al. [37]RQ2 What are the most important applied ML techniques for predictingobesity issues?Researchers have drawn from various techniques to build predictiveand prognostic models for biomedical applications. In addition to thelogistic and Cox regression models that are most often utilized [39], MLtechniques have generally shown promising potential [40]. In particular, when used as an algorithmic framework, ML can provide insightinto data collections, facilitate the development of inferences, and evenderive knowledge from findings. Therefore, ML approaches have alreadybeen applied across for a variety of prognostic and diagnostic purposes[41]. ML algorithms have also been applied across various health andhealthcare domains to predict the development or presence of particularhealth conditions based on pre-determined characteristics [42]. ML hasalso been particularly utilized in obesity research [4346]. However,some researchers caution that ML describes a wide-ranging variety oftechniques, which can be characterized only broadly depending onwhether their learning phases are supervised (i.e., whether a specificSun et al. [51]Al-Raddadi et al.[52]Socio-economic statusPovertyUnemploymentBehavioral factorsSugary drinks consumptionFruits and vegetable consumptionBuilt environmentPrevalence of physical activitySupermarket accessibilityPersonal lifestyle driversUnhealthful dietLack of physical activityFamily reasonsPerceived stress of workIrregular lifeSocietal driversPrevalence of fast foodTransport and/or new technologyLack of facilitiesMisleading ads related to health and/or consumptionSociodemographic characteristicsDemographics of ageDemographics of ethnicityIndividual and collective education levelsRelative living statusAnnual household income, as calculated per capitaIndividual behavioral factorsCurrent habits and/or history of smokingCurrent habits and/or history of excessive drinkingCurrent habits and/or history of regular, excessive red meatintakeSmoking statusEthnicityPhysical activity6(continued on next page)M. Safaei et al.Computers in Biology and Medicine 136 (2021) 104754Table 5 (continued )Author (s)Tulp et al. [53]Gomez-Llorente et al.[32]Hu et al. [8]Kadouh & Acosta [5]Lecube et al. [54]Nikookar et al. [55]Yang et al. [56]Wang et al. [57]Cois and Day [58]Sartorius et al. [36]Table 5 (continued )Factor(s)SmokingAlcohol consumptionSoft drink consumptionLow physical activityAuthor (s)Factor(s)Shi et al. [59]Alcohol consumptionCigarette smokingHours of sleep (per night/sleeping period)Free-time exerciseGenetic influencesEthnic differencesGestational weight and/or intrauterine factorsDietSocio-economic statusLevel of physical activitySleepParental determinantsAssortative mating (e.g., with similar phenotypes)Parental traits (e.g., age and education at subject birth andduring childhood)Body image and/or perceptions thereofSleep habits and/or conditionsDegree of physical activity/evidence of sedentary lifestyleLevels of alcohol consumptionEducational level and/or qualificationsAng et al. [60]Over nutritionGenetic factorsSmoking habitAlcohol consumptionBiological factorsGenetics of obesityBrain-gut axis, including gut microbiomePrenatal determinantsExperience of pregnancy or current pregnancyMenopauseNeuroendocrine conditionsUse of medicationsPresence of physical disability and/or disabilitiesPresence of virusesEnvironmental factorsObesogenic environmentSurrounding society and culture(s)Chemicals present in current and/or childhoodenvironmentBehavioral factorsFrequent caloric intake and/or continuous eating patternsSedentary lifestyle and/or physical inactivityInsufficient or deficient sleepPast or present smoking habitsPhysical activityNutritional habitsConsumption of unhealthy beverages, either soft drinks oralcoholConsumption of alcohol (e.g., wine, beer, and/or otheroptionswith high alcohol content)Sleep patternDifficulties following healthy dietHistory of parental obesityIndividual childhood obesityMenopauseEnergy intakeOver-large serving sizes for particular foods, includinggrains(bread & cereal), meat, and fatsPhysical activitySleep durationSocial isolationCurrent cigarette smokerPhysically inactiveConsumption of fast food on regular basisExcess consumption (abuse) of alcoholUse of illicit drugsSmokingDrinkingDietSleep (measured as hours per night)SmokingUse of alcoholExercise frequencyIndividual FactorsGenetic factors (e.g., sex, ethnicity)Socio-economic factors (e.g., income and education)DepressionSocial FactorsFamily influences (e.g., marriage)Peer influencesLifestyle/BehavioralFood consumption (e.g., energy intake)Physical activityEnvironmental FactorsCommunity characteristics (e.g., rural urban, access tounhealthy food options, crime)Economic (e.g., cost, price, trade)State policiesMarketingBressan et al. [61]Cheng and Furnham[30]Michels et al. [30]Specific traits (e.g., extraversion, conscientiousness)Past or present psychological distressLacking or insufficient physical activity and exerciseChronic stressDietSleepPhysical activityFig. 5. Factors often leading to adult overweightness/obesity.algorithm uses outcome data for training). Supervised ML methodsinclude classifiers, while unsupervised ones include clustering andsemi-supervised methods include options such as label propagation[47].Even before the proliferation of ML techniques, other so-calledexpert systems (such as early attempts with artificial intelligence, orAI) had been utilized in the analysis of medical images and scans or eventhe detection of certain medical or physiological abnormalities [42].However, ML represents a powerful new set of fine-tuned algorithmswith a considerable collective capability to learn, adapt, predict, andanalyze data, which can all introduce unprecedented precision to7M. Safaei et al.Computers in Biology and Medicine 136 (2021) 104754obesity predictions, among other conditions [44]. Therefore, ML hasbeen applied to questions and problems in obesity-based research withincreasing frequency.This study identified the ML techniques used from the overall list ofselected articles and the risk factors considering obesity (Table 6). Forexample, Singh and Tawfik [42] investigated numerous multivariateregression algorithms and multi-layer perceptron feed forward artificialneural networks (MLFFNN) on a dataset obtained from a millenniumcohort study (MCS) and acquired over 93.4% accuracy in their attemptsto predict teenage BMI from existing BMI values. These results areencouraging, particularly their achievement of prediction accuracy over90%.Uar et al. [48] estimated individual percentages of body fat usinghybrid machine learning algorithms, such as MLFFNN, support vectormachine regression model (SVM), and decision tree (DT) regression.This study also used real data sets, which comprised 13 anthropometricmeasurements of actual individuals.Herein, estimations could be made with a correlation value of R =0.79 regarding any single data set from among the 13 anthropometricmeasurements. These results demonstrate that a developed ML systemcould also be used in practice to estimate body fat percentagesaccurately.Dugan et al. [44] conducted a study that used ML techniques for theprediction of early childhood obesity. This study applied six differentmachine learning approaches (random tree, random forest, J48, ID3,Nave Bayes, and Bayes trained) and collected data through a pediatricclinical decision support system called CHICA. The results of this studyafter training and evaluation showed that the ID3 model trained usingthis CHICA dataset offered the best overall performance: 85% accuracyand 89% sensitivity.Lingren et al. [62] developed their rule- and ML-based algorithms toidentify children with severe, early-onset obesity accurately. Their results revealed that the rule-based algorithm offered optimal performance, yielding 0.895 (CCHMC) and 0.770 (BCH).Fernandez-Navarro et al. [63] investigated obesity-driven interactions between serum free fatty acids and fecal microbiota usingan ML algorithm. Classifications were managed using DTs, which drovethe performance of statistical analyses and the identification of predictive factors for obesity. They found that Serum eicosatetraenoic andgender are the most significant variables identified, with 100% and 80%significance, respectively. Lazzarini et al. [64] applied an ML approachto their identification of new biomarkers for tracking the development ofknee-based osteoarthritis in overweight and obese women. Rankedguided iterative feature elimination (RGIFE), which is an ML heuristic,was applied to select biomarkers that demonstrated high predictivepower. The models of Lazzarini et al. [64] also offered high performance(AUC >0.7) despite using relatively few variables.Multiple researchers have also shown that, overweightness andobesity are outcomes of energy imbalances in the human body. Manycommon treatments, such as special diets, balanced caloric intake, andenhanced activity, are intended to treat such imbalances. Pouladzadehet al. [65] applied deep convolutional neural networks (DCNN) in anattempt to classify over 10,000 high-resolution images of food. The results obtained showed 99% accuracy in recognizing single portions offood, which is close to appropriate portions for those attempting tocombat and/or avoid high BMI.Farran et al. [66] performed a study predicting future risks of type 2diabetes among the Kuwait population by using three models based onthree techniques: LR, k-nearest neighbors (k-NN), and SVMs withfive-fold cross-validation. Their techniques outperformed most othercommonly used methods, LR and otherwise.Jindal et al. [67] employed the ensemble prediction model to propose an ensemble ML approach for predicting obesity based on fourmain determinants: age, height, weight, and BMI. They utilized RandomForest (RF), generalized linear model, and partial least square, and ultimately, the average predicted values were 89.68% accurate.Dunstan et al. [68] implemented three different ML algorithms(SVM, RF and extreme gradient boosting) for nonlinear regression inpredicting obesity at the national level. Their method was validatedconsidering its absolute prediction error and the proportion of surveyedcountries, in which the prevalence of obesity was predicted satisfactorily. Their study forecasted that flours and baked goods, dairy-productcheeses, and sugar-sweetened carbonated drinks were the food categories that most closely and accurately predicted the prevalence ofobesity.Taghiyev et al. [69] developed a two-stage classification model usingDT and logistic regression (LR) to identify effectively the causes ofTable 6Potential factors that influence and/or cause obesity.Author (s)Machine Learning technique(s)Risk Factor(s)Singh & Tawfik[42]Multi-layer perceptron feedforward artificial neuralnetworks (MLFFNN)MLFFNN, Support VectorMachine (SVM) and DecisionTree Regression (DT)Random Tree (RF), J48, ID3,Nave Bayes NB), and BayestrainedArtificial Neural Networks(ANN)Rule-based and ML-basedalgorithmsDecision Trees (DT)Body Mass Index (BMI)Uar et al. [48]Dugan et al. [44]Fergus et al. [89]Lingren et al.[62]FernandezNavarro et al.[63]Lazzarini et al.[64]Pouladzadehet al. [65]Farran et al. [66]Selya & Anshutz[47]Jindal et al. [67]Pleuss et al. [90]Dunstan et al.[68]Gupta et al. [91]Taghiyev et al.[69]Machorro-Canoet al. [70]de MouraCarvalho et al.[92]Gerl et al. [93]Montanez et al.[94]Wiechmann et al.[95]Pang et al. [96]Pereira et al. [97]Rajput et al. [98]Kibble et al. [99]Scheinker et al.[100]Zheng &Ruggiero [101]Wang et al. [102]Ranked Guided Iterative FeatureElimination (RGIFE)Deep convolutional neuralnetworks (CNN)Logistic Regression (LR), Knearest Neighbors (K-NN), andSupport Vector Machines (SVM)SVMRFML and 3D image processingSVM, RF and Extreme GradientBoostingRecurrent Neural Network(RNN) architecture with LongShort-term Memory (LSTM)DT and LRJ48 algorithmDTLasso ModelGradient Boosting (GB),Generalized Linear Model,Regression Trees (RT), KNN,SVM, RF, and MLFFNNC4.5Body Fat Percentage (BFP)Prediction of obesityBMIPrediction of obesityFatty acidsKnee osteoarthritisFood and energy Intake forcombatting obesityBMI and type 2 diabetesDietary and physicalactivityBMIBMI, Waist Circumference(WC), or HipCircumference (HC)Prediction of obesityBMICauses of obesityPrediction, prevention,and detection of obesityTackling obesityBMI, WC, waist-hip ratio(WHR), and BFPPrediction of obesityDiagnosis of obesityXGBoost model predictionsLogistic Regression (LR), NB, DT,KNN, RF, and AdaBoostDLGroup factor analysis (GFA)GB and multivariate LRPrediction of obesityPrediction of obesityPrediction of obesityPrediction of obesityBMIDT, Weighted KNN, and ANNPrediction of obesitySVM, KNN, and DTPrediction of obesity8M. Safaei et al.Computers in Biology and Medicine 136 (2021) 104754obesity among females aged 18 years and above in the region of Turkey.Their proposed hybrid system achieves 91.4% accuracy, which is betterthan other classifiers (i.e., 4.6% and 2.3% higher than the performanceof LR and DT, respectively).Machorro-Cano et al. [70] offered PISIoT, which was an ML- andIoT-based smart health platform intended to prevent, detect, and treatobesity. They applied the Weka API and J48 ML algorithms to identifycritical variables regarding obesity and classify patients. Their investigation also presented a case study regarding the prevention ofmyocardial infarction in obese elderly patients through the monitoringof biomedical variables to validate the proposed PISIoT platform.The existing applications of ML techniques to predict instances ofobesity are presented in Fig. 6. This figure shows that ML techniqueswere categorized in accordance with single and hybrid methods.These results demonstrate that the artificial neural networks (ANNs)approach was the most often used method in previous studies. ANNs arerelated to ML methods [42]. ANNs can solve several types ofmulti-variate, nonlinear problems when presented with the appropriatetraining algorithm and useful amounts of data [71]. Different ANNs havealso been employed to predict the presence of disease and solve othercomplex problems, though only those based on a set of known parameters. The ANN architecture entails at least three layers: one for input,one hidden (though this can also include sub-layers), and one for output[72]. Every layer includes multiple nodes responsible for transmittingimportant information between layers despite the impossibility ofneither lateral nor feedback connection [73]. ANNs have attractedconsiderable interest over the last decade due to, their use as predictivemodels and for their possibilities regarding pattern recognition.hypertension and stroke, type 2 diabetes (mellitus), sleep apnea, andosteoarthritis [74]. Table 7 offers a summary of known obesity-relateddiseases and conditions. Neurodegenerative diseasesNeurodegenerative diseases or disorders in which the central or peripheral nervous system degenerates progressively, are another growingpublic health concern. The data from various studies have revealed aworldwide increase in co-occurrences of neurodegenerative diseases andobesity [75]. Moreover, evidence suggests correlations between adultobesity and patients development of specific neurodegenerative diseases such as Alzheimers disease (AD) and Parkinsons disease (PD),due to the correlating factor of type 2 diabetes mellitus [76]. Dementia,which has correctly been characterized as a chief cause of cognitivefunction impairment in older adults, may also be linked to these factors[77]. Mazon et al. [78] examined associations among various neurodegenerative diseases, focusing on AD, PD, and individual metabolicchanges. Their results showed that adult obesity can kickstart thedevelopment of neurodegenerative diseases, while diet-induced metabolic dysfunctions can aggravate existing conditions. Profenno et al.[79] confirmed that adult obesity and diabetes increase the risk of patients for AD. Midlife and in old age, increased risks for AD are consistentwith AD pathogenesis, beginning several years before the clinical onsetof the condition. Cardiovascular diseaseObesity takes a considerable toll on the human cardiovascular (CV)system. In particular, adult obesity tends to worsen several key riskfactors. Factors and conditions, such as hypertension, coronary heartdisease, atrial fibrillation, and outright heart failure, are all exacerbatedin patients with obesity as a pre-existing condition [8082].RQ3: What are the major disorders/diseases associated with obesitybased on the work of previous studies?The medical consequences of adult obesity are numerous and complex. One of the most concerning is the prevalence of obesity as a publichealth crisis. Another is that obesity increases the risks of individuals forother primary lifestyle diseases, which include coronary heart disease, Prostate diseasesFig. 6. ML techniques for obesity prediction.9M. Safaei et al.OtherCarcinogenesisandinfertilityMetabolicsyndromePneumoniaDigestiveDiseasesMusculoskeletalDisordersMentalDisordersCancerNon communicablediseasesDiabetesOraldiseasesPhenotypesAutoimmunediseasesRespiratorydiseasesProstatediseasesHyper Tension10Computers in Biology and Medicine 136 (2021) 104754[110][78][79][82][81][84][128][12][120][85][12][86][119][80][129][88][106][122][104][121][124][109][125][114][123][130][131][132][133][111][118][134][112][135][136][117][115][113][126][137][138][137][139]NeurodegenerativediseasesCardiovasculardiseases(CVDs)Table 7Major diseases that are associated with obesity in the previous studies.M. Safaei et al.Computers in Biology and Medicine 136 (2021) 104754Prostatitis, or inflammation of the prostate in men, is a common butpoorly-defined medical condition. Its many causes and different durations also lead to a lack of uniform diagnostic criteria and clear approaches to treatment. However, prostatitis can be found in 10%14% ofmen, regardless of age, socioeconomic status, or racial and ethnic origin,and nearly 50% of men will experience this condition at some point intheir lives [83]. Parikesit et al. [84] also found that obesity is a riskfactor for various versions of prostate diseases, including benign prostate hyperplasia (BPH) and prostate cancer (PCa).between obesity and short-term mortality among patients hospitalizedwith pneumonia. However, these connections had minimal to no impacton mortality considering many other types of infections.Table 7 and Fig. 7 reveal that obesity demonstrated several connections to other types of diseases. Numerous studies included in thisSLR have discussed the adverse effects of obesity on various cardiovascular conditions and diseases [8082,104,106,110121]. For instance,patients who were overweight or obese displayed a higher incidence ofcardiovascular diseases of almost every kind compared with those patients with normal BMIs and weights [117]. Thus, the research suggeststhat CVD preventive strategies should also target obesity, and thesestrategies must be circulated widely to address underlying conditions atthe popular level. Researchers conclude that this kind of preventiveapproach would reduce not only workplace issues, such as employeeinsurance rates and absenteeism for medical leave, but also large socialissues, including medical costs (hospital stays, treatments, and drugs)that currently burden numerous healthcare systems worldwide.The twin epidemics of obesity and diabetes have combined to form amajor global health crisis. Several studies collected herein indicate that,diabetes was also one of the most commonly-considered comorbiditiesof obesity [104,106,111,114,118,119,121126]. In a related vector,researchers also estimated that as much as 90% of type 2 diabetes can beattributed to high BMI or excessive weight of patients [126]. Respiratory diseasesRespiratory systems and respiratory diseases are also adverselyimpacted by obesity. Obesity can worsen existing conditions or triggernew ones. More specifically, several common respiratory problems, suchas asthma and obstructive sleep apnea, are more prevalent in obesechildren than in peers with healthy weights [85]. AutoimmunityResearchers have also sought further knowledge regarding relationships between adult obesity and autoimmunity disorders. Studieshave already indicated to the possibility that this confluence is impactedby a combination of environmental factors. Of the ones alreadyconsidered, strong evidence points toward the adverse impact of obesityon many immune-mediated conditions because they develop andworsen. These conditions include rheumatoid arthritis (RA), systemiclupus erythematosus (SLE), inflammatory bowel disease (IBD), multiplesclerosis (MS), type-1 diabetes (T1D), and thyroid auto-immunity (TAI),especially Hashimoto thyroiditis (HT) [86].5. Discussion and conclusionObesity is a preventable but all-too-common health condition thathas recently developed into a global epidemic, impacting public healthat multiple levels. This SLR and the collected primary works reveal thatseveral studies have determined that adult obesity and even beingoverweight are unmistakably associated with various co-morbidities,which include CVD, cancers, and various chronic conditions. As aninitial approach to the studies already conducted in this area, the currentSLR has presented an outline of recently published articles related toobesity or being overweight, particularly in connection to ML methods,for diagnosis and/or prevention. Herein, a systematic approach wasused to explore three defined research questions using articles that fitseveral criteria and were evaluated using systematic methods.The outlined results herein indicate that many factors cause and AutoimmunityNon-communicable diseases (NCD) are another type of conditionadversely impacted by obesity, particularly the earlier identified cardiovascular conditions as well as type 2 diabetes, osteoarthritis, andsome categories of cancer. In the Middle East specifically, NCDs are theforemost cause of mortality, accounting for 60% of all deaths annually[87]. Kilpi et al. [88] examined trends and predictions regarding obesityin nine countries across the Middle East and, found high and increasingobesity rates in men and women for most of the studied countriesstudied. They recommended that diagnoses of strokes and type 2 diabetes would be decreased with even minor reductions in collective levelsof obesity. DiabetesOverweightness and obesity are also well-documented as increasingthe risk of diabetes, particularly type 2 diabetes mellitus [103]. de Oliveira et al. [104] confirmed that diabetes occurs with high incidence inobese patients. aTbares2017health [105] indicated that diabetes followed obesity in 2015 as the second leading cause of BMI related deaths,contributing to 0.6 million mortalities worldwide. Similarly, Lette et al.[106] calculated that healthcare costs attributable to overweight-relateddiseases could account for as much as 26% of spending, with maincontributors to this number being diabetes, followed by endometrialcancer and then osteoarthritis. PneumoniaObesity increases mortality rates for many disorders and the susceptibility of patients to various everyday infections [107]. The mechanisms that could be underlying this connection are not yet clear. Thus,these mechanisms could be related to deregulation of the immune system, and by extension, to co-morbidities either caused or exacerbated byobesity [108]. For instance, Atamna et al. [109] found connectionsFig. 7. Specific types of diseases related to obesity.11Computers in Biology and Medicine 136 (2021) 104754M. Safaei et al.exacerbate overweightness and obesity, particularly among adults. ThisSLR determined that certain factors are often considered crucial parameters and leading factors of obesity. This study then reviewed MLtechniques used to predict obesity. This review indicated that ML iscurrently continuously used in researching obesity. However, this SLRalso reveals that only limited research has reported using ML specificallyfor detecting obesity. This aspect remains a promising research areabecause ML techniques can provide far more robust rates of predictionaccuracy than those achieved using simple techniques, including statistical methods, such as linear regression. Uar et al. [48] indicatedthat, ML methods are also preferable for performance alone. The use ofgeneral equations to estimate body fat percentages is already a majorindicator of the effective performance of ML in this area.Next, specific ML approaches can be categorized as either single orhybrid method based on the trends identified in this SLR. As of thiswriting, the ANN approach is the most often applied ML method in theexisting literature. Finally, this SLR also reviewed certain types of diseases related to obesity. The results confirmed that obesity is a healthcare concern and epidemic worldwide and is also well documented as arisk for increasing rates of various other conditions.Therefore, obesity deserves serious consideration and attention frompolicymakers, healthcare providers, and researchers alike. Obesity prevention must be multifaceted and should actively involve stakeholdersat different levels. From potential areas of policy to the development andimplementation of these policies, approaches should account for peoples home environments and broad, society-level views of socioeconomic environments. However, many barriers that prevent strategizingon the level of policy alone may be encountered. Instead, the reductionand prevention of obesity will considerably depend on individual lifestyle changes. In this case, further research on motivations for individualand societal behavioral changes is crucial.attributed to the expected report on validated findings by peer-reviewedjournal papers, which also have the most significant influence on thefield [127]. The second limitation is the restricted search for articles toonly six online databases: i.e., Science Direct, SpringerLink, IEEE Explorer, Taylor and Francis Online, ACM Digital Library, MDPI, and NCBI.Nevertheless, these databases were selected because they are among themost extensively-used in the world [46], and each one indexes journalsand articles from any discipline associated with the review, namelymedicine, engineering, and computer science. Thus, articles publishedin these fields, as well as those in healthcare, discipline associated.Additionally, locating all published research during the 10 yearsunder investigation cannot be guaranteed with the growing amount ofresearch on obesity. This comprehensive exploration still illustrates thecurrent status of research on obesity using ML methods. However, futurestudies should consider substantially expanded approaches, such asadditional databases or consideration of conceptualized and unpublished research and peer-reviewed scholarship.Finally, this study only introduced and reviewed ML techniquesbecause they are used for obesity prediction. Therefore, future workshould evaluate the robustness and weaknesses of each technique usedin this area.8. Further workThe present study is intended as a step toward effectively understanding obesity and its identification, investigation, and treatment. Thecollected findings are promising, but additional research is required toincrease understanding of this disease. For instance, one of the primaryobjectives of this study was to uncover the ML techniques already oftenapplied to predict obesity. Therefore, future researchers should test eachML algorithm for accuracy and robustness when attempting to expandthe research in this field. Moreover, this study briefly examined the mostinfluential factors that cause or worsen obesity. However, this complexarea demands additional further research, particularly because it couldalso refocus the other influential factors that have already been determined to result in obesity. Finally, future work should continue to focuson developing effective and safe interventions to combat the spread ofobesity and related health risks, conditions, and diseases.6. Clinical implications of the studyAn important clinical implication of the findings is the identificationof ML techniques that are most often used successfully in prior studies.ML techniques provide substantially more robust prediction accuracyrather than using simple techniques, such as linear regression, or otherstatistical methods. Overall, ML techniques, such as neural network, DT,RF, and Deep Learning, are some of the most effective approachesenabling the early detection and clinical management of obesity.Declaration of competing interestNone Declared.7. Limitations of the studyAcknowledgementsFirst, this study included peer-reviewed journal papers and excludedconference articles, books, and unpublished full-text papers, which bothfollow best practices in academic publishing but could have also limitedits access to other research and results. However, this decision isThis research was supported and funded by the Universiti Kebangsaan Malaysia, under High Impact Research Grant: DIP-2018-041.Appendix. Primary studiesTable Appendix: Primary studiesStudyIDStudy TitleAuthorsS1S2S3S4S5S6S7S8S9Factors Affecting Adult Overweight and Obesity in Urban ChinaObesogenic environmental factors of adult obesity in China: a nationally representative cross-sectional studyPrevalence of overweight, obesity, abdominal obesity and obesity-related risk factors in southern ChinaChildrens Body composition and Stressthe ChiBS study: aims, design, methods, population and participation characteristicsPhytocompounds as potential agents to treat obesity-cardiovascular ailmentsObesity and asthma: a missing linkSocial components of the obesity epidemicMultifactorial influences of childhood obesityCurrent paradigms in the etiology of obesityIshida et al. [29]Zhang et al. [13]Hu et al. [8]Michels et al. [140]Meriga et al. [33]Gomez-Llorente et al. [32]Bressan et al. [61]Ang et al. [60]Kadouh, H.C. and Acosta, A[5].(continued on next page)12M. Safaei et al.Computers in Biology and Medicine 136 (2021) 104754(continued )Table Appendix: Primary studiesStudyIDStudy TitleAuthorsS10S11S12S13S14Overweight/obesity and associated cardiovascular risk factors in sub-Saharan African children and adolescents: a scoping reviewDeterminants of obesity and associated population attributability, South Africa: Empirical evidence from a national panel surveyBiomedical, psychological, environmental and behavioural factors associated with adult obesity in a nationally representative sampleImpact of Disadvantaged Neighborhoods and Lifestyle Factors on Adult Obesity: Evidence From a 5-Year Cohort Study in AustraliaEstimating neighborhood-level prevalence of adult obesity by socio-economic, behavioral and built environment factors in New YorkCityObesity as a self-regulated epidemic: coverage of obesity in Chinese newspapersAdult obesity and mid-life physical functioning in two British birth cohorts: investigating the mediating role of physical inactivityFactors Accounting for Obesity and Its Perception among the Adult Spanish Population: Data from 1,000 Computer-Assisted TelephoneInterviewsThe prevalence of adult obesity in Africa: A meta-analysisObesity trends and risk factors in the South African adult populationYoung adult risk factors for cancer: obesity, inflammation, and sociobehavioral mechanismsPrevalence of overweight and obesity and some associated factors among adult residents of northeast China: a cross-sectional studyFactors Associated with Obesity: A CaseControl Study of Young Adult Singaporean MalesThe prevalence of obesity and overweight, associated demographic and lifestyle factors, and health status in the adult population ofJeddah, Saudi ArabiaOverweight, Obesity, and Its Associated Factors in Adult Women Referring to Health Centers in Shiraz in 20132014Personality traits, education, physical exercise, and childhood neurological function as independent predictors of adult obesityA Machine Learning Approach for Predicting Weight Gain Risks in Young AdultsEstimation of body fat percentage using hybrid machine learning algorithmsMachine learning techniques for prediction of early childhood obesityA machine learning approach to measure and monitor physical activity in children to help fight overweight and obesityDeveloping an algorithm to detect early childhood obesity in two tertiary pediatric medical centersExploring the interactions between serum free fatty acids and fecal microbiota in obesity through a machine learning algorithmChoukem et al. [31]Sartorius et al. [36]Cheng et al. [38]Keramat et al. [49]Sun, Y. et al. [37]S15S16S17S18S19S20S21S22S23S24S25S26S27S28S29S30S31S32S35S36S37S38S39S40S41A machine learning approach for the identification of new biomarkers for knee osteoarthritis development in overweight and obesewomenFood calorie measurement using deep learning neural networkUse of Non-invasive Parameters and Machine-Learning Algorithms for Predicting Future Risk of Type 2 Diabetes: A Retrospective CohortStudy of Health Data from KuwaitMachine Learning for the Classification of Obesity from Dietary and Physical Activity Patterns, Advanced Data Analytics in HealthObesity prediction using ensemble machine learning approaches, Recent Findings in Intelligent Computing TechniquesA machine learning approach relating 3D body scans to body composition in humansPredicting nationwide obesity from food sales using machine learningObesity Prediction with EHR Data: A deep learning approach with interpretable elementsA Hybrid Approach Based on Machine Learning to Identify the Causes of ObesityPISIoT: A machine learning and IoT-based smart health platform for overweight and obesity controlS42Using Machine Learning for Evaluating the Quality of Exercises in a Mobile Exergame for Tackling Obesity in ChildrenS43S44S45S46S47S48S49S54S55S56S57S58S59S60S61S62S63Machine learning of human plasma lipidomes for obesity estimation in a large population cohortMachine learning approaches for the prediction of obesity using publicly available genetic profilesIdentifying discriminative attributes to gain insights regarding child obesity in hispanic preschoolers using machine learning techniquesUnderstanding Early Childhood Obesity via Interpretation of Machine Learning Model PredictionsObesity Related Disease Prediction from Healthcare Communities Using Machine LearningObesity and Co-Morbidity Detection in Clinical Text Using Deep Learning and Machine Learning TechniquesAn integrative machine learning approach to discovering multi-level molecular mechanisms of obesity using data from monozygotic twinpairsIdentification of Factors Associated with Variation in US County-Level Obesity Prevalence Rates Using Epidemiologic vs MachineLearning ModelsA review of machine learning in obesityUsing machine learning to predict obesity in high school studentsMachine Learning-Based Method for Obesity Risk Evaluation Using Single-Nucleotide Polymorphisms Derived from Next-GenerationSequencingClinical relevance of adipokinesThe impact of obesity on neurodegenerative diseasesMeta-analysis of Alzheimers disease risk with obesity, diabetes, and related disordersObesity and prevalence of cardiovascular diseases and prognosisthe obesity paradox updatedAn overview and update on obesity and the obesity paradox in cardiovascular diseasesThe impact of obesity towards prostate diseasesAstrocytes and endoplasmic reticulum stress: A bridge between obesity and neurodegenerative diseasesImpact of obesity and the obesity paradox on prevalence and prognosis in heart failureObesity phenotypes and their paradoxical association with cardiovascular diseasesObesity and common respiratory diseases in childrenS64S65S66S67S68S69S70Obesity and cardiovascular diseases: implications regarding fitness, fatness, and severity in the obesity paradoxObesity in autoimmune diseases: not a passive bystanderObesity phenotypes, diabetes, and cardiovascular diseasesAssociation of central obesity with the incidence of cardiovascular diseases and risk factorsRelationship between obesity and oral diseasesAlarming predictions for obesity and non-communicable diseases in the Middle EastHealth care costs attributable to overweight calculated in a standardized way for three European countriesS33S34S50S51S52S5313Sun, S. et al. [51]Pinto Pereira et al. [50]Lecube et al. [54]Tulp et al. [53]Cois and Day [58]Yang et al. [56]Wang et al. [57]Shi et al. [59]Al-Raddadi et al. [52]Nikookar et al. [55]Cheng and Furnham [30]Singh and Tawfik [42]Uar et al. [48]Dugan et al. [44]Fergus et al. [89]Lingren et al. [62]Fernandez-Navarro et al.[63]Lazzarini et al. [64]Pouladzadeh et al. [65]Farran et al. [66]Selya and Anshutz [47]Jindal et al. [67]Pleuss et al. [90]Dunstan et al. [68]Gupta et al. [91]Taghiyev et al. [69]Machorro-Cano et al. [70,70]de Moura Carvalho et al.[92]Gerl et al. [93]Montanez et al. [94]Wiechmann et al. [95]Pang et al. [96]Pereira et al. [97]Rajput et al. [98]Kibble et al. [99]Scheinker et al. [100]DeGregory et al. [141]Zheng and Ruggiero [101]Wang et al. [102]Blher and journal [110]Mazon et al. [78]Profenno et al. [79]Lavie et al. [82]Elagizi et al. [81]Parikesit et al. [84]Martin-Jimenez et al. [128]Lavie et al. [116]Vecchie et al. [120]Xanthopoulos and Tapia[85]Lavie et al. [12]Versini et al. [86]Piche et al. [119]Barroso et al. [80]Sede and Ehizele [129]Kilpi et al. [88]Lette et al. [106](continued on next page)M. Safaei et al.Computers in Biology and Medicine 136 (2021) 104754(continued )Table Appendix: Primary studiesStudyIDStudy TitleAuthorsS71S72Obesity, lifestyle risk-factors, and health service outcomes among healthy middle-aged adults in CanadaDirect healthcare cost of obesity in Brazil: an application of the cost-of-illness method from the perspective of the public health system in2011The burden of overweight and obesity on long-term care and Medicaid financingEconomic costs of obesity in Thailand: a retrospective cost-of-illness studyHow obesity impacts outcomes of infectious diseasesObesity, diabetes, and cardiovascular diseases: a compendiumObesity: how much does it matter for female pelvicorgan prolapse?How western diet and lifestyle drive the pandemic of obesity and civilization diseasesObesity, Metabolism, 2014. Diabetes and cancer: two diseases with obesity as a common risk factorOverweight, obesity and related non-communicable diseases in Asian Indian girls and womenNew national data show alarming increase in obesity and noncommunicable chronic diseases in ChinaObesity as a risk and severity factor in rheumatic diseases (autoimmune chronic inflammatory diseases)Obesity-related digestive diseases and their pathophysiologyAn integrated framework for the prevention and treatment of obesity and its related chronic diseasesEndocrine disruptors leading to obesity and related diseasesObesity and respiratory diseasesStress and obesity as risk factors in cardiovascular diseases: a neuroimmune perspectiveThe prevalence and trends of overweight, obesity and nutrition-related non-communicable diseases in the Arabian Gulf StatesHigh rates of obesity and non-communicable diseases predicted across Latin AmericaManagement of cardiovascular diseases in patients with obesityObesity and the obesity paradox in cardiovascular diseasesThe association of periodontal diseases with metabolic syndrome and obesityObesity and diabetes: an updateAlter et al. [122]de Oliveira et al. [104]S73S74S75S76S77S78S79S80S81S82S83S84S85S86S87S88S89S90S91S92S93References[14][1] Salvador Camacho, Andreas Ruppel, Is the calorie concept a real solution to theobesity epidemic? Glob. Health Action 10 (1) (2017) 1289650.[2] Sadaf Ibrahim, Zuneera Akram, Aisha Noreen, Mirza Tasawer Baig,Samina Sheikh, Ambreen Huma, Aisha Jabeen, Muneeza Lodhi, ShahzadaAzam Khan, Hudda Ajmal, Uzma Shahid, Nayel Syed, Overweight and obesityprevalence and predictors in people living in Karachi, J. Pharmaceut. Res. Int.(2021) 194202.[3] D.S. Akram, A.V. Astrup, T. Atinmo, J.L. Boissin, G.A. Bray, K.K. Carroll,P. Chitson, C. Chunming, W.H. Dietz, J.O. Hill, E. Jequier, C. Komodiki,Y. Matsuzawa, W.F. Mollentze, K. Moosa, M.I. Noor, K.S. Reddy, J. Seidell,V. Tanphaichitr, R. Uauy, P. Zimmet, Obesity: Preventing and Managing theGlobal Epidemic. Number, 2000, p. 894.[4] Chunlan Zhang, Jingjing Zhang, Zhenqi Liu, Zhiguang Zhou, More than an antidiabetic bariatric surgery, metabolic surgery alleviates systemic and localinflammation in obesity, Obes. Surg. 28 (11) (2018) 36583668.[5] Hoda C. Kadouh, Andres Acosta, Current paradigms in the etiology of obesity,Tech. Gastrointest. Endosc. 19 (1) (2017) 211.[6] Ellen P. Williams, Marie Mesidor, Karen Winters, Patricia M. Dubbert, SharonB. Wyatt, Overweight and Obesity: Prevalence, Consequences, and Causes of aGrowing Public Health Problem, 2015.[7] Syahrul Sazliyana Shaharir, Abdul Halim Abdul Gafor, Mohd Shahrir MohamedSaid, C. Norella, T. Kong, Steroid-induced diabetes mellitus in systemic lupuserythematosus patients: analysis from a Malaysian multi-ethnic lupus cohort, Int.J. Rheum. Dis. 18 (5) (2015) 541547.[8] Lihua Hu, Xiao Huang, Chunjiao You, Juxiang Li, Kui Hong, Ping Li, Yanqing Wu,Qinhua Wu, Zengwu Wang, Runlin Gao, Huihui Bao, Xiaoshu Cheng, Prevalenceof overweight, obesity, abdominal obesity and obesity-related risk factors insouthern China, PloS One 12 (9) (2017), e0183934.[9] Natharnia Young, , Ixora Kamisan Atan, Rodrigo Guzman Rojas, Hans Peter Dietz,Obesity: how much does it matter for female pelvic organ prolapse? Int.Urogynecol. J. 29 (8) (2018) 11291134.[10] Hlya akmur, Introductory chapter: unbearable burden of the diseases - obesity,in: Obesity. IntechOpen, 2020.[11] Stephan C. Bischoff, Yves Boirie, Tommy Cederholm, Michael Chourdakis,Cristina Cuerda, Nathalie M. Delzenne, Nicolaas E. Deutz, Denis Fouque,Laurence Genton, Carmen Gil, Berthold Koletzko, Miguel Leon-Sanz,Raanan Shamir, Joelle Singer, Pierre Singer, Nanette Stroebele-Benschop,Anders Thorell, Arved Weimann, Rocco Barazzoni, Towards a multidisciplinaryapproach to understand and manage obesity and related diseases, Clin. Nutr. 36(4) (2017) 917938.[12] Carl J. Lavie, Paul A. McAuley, Timothy S. Church, Richard V. Milani, StevenN. Blair, Obesity and cardiovascular diseases: implications regarding fitness,fatness, and severity in the obesity paradox, J. Am. Coll. Cardiol. 63 (14) (2014)13451354.[13] Xiao Zhang, Mei Zhang, Zhenping Zhao, Zhengjing Huang, Qian Deng,Yichong Li, An Pan, Li Chun, Zhihua Chen, Maigeng Zhou, Chao Yu, Alfred Stein,Peng Jia, Limin Wang, Obesogenic environmental factors of adult obesity in[15][16][17][18][19][20][21][22][23][24][25][26][27]14Yang and Zhang [121]Pitayatienanan et al. [124]Atamna et al. [109]Scherer and Hill [125]Young et al. [9]Kopp et al. [114]Garg et al. [123]Chopra et al. [130]Wang et al. [131]Gremese et al. [132]Nam and liver [133]Dietz et al. [111]Petrakis et al. [118]Zammit et al. [134]Ippoliti et al. [112]Ng et al. [135]Webber et al. [136]Lavie et al. [117]Lavie et al. [115]Jepsen et al. [113]Verma et al. [126]China: a nationally representative cross-sectional study, Environ. Res. Lett. 15 (4)(2020), 044009.M. Simmonds, A. Llewellyn, C.G. Owen, N. Woolacott, Predicting adult obesityfrom childhood obesity: a systematic review and meta-analysis, Obes. Rev. 17 (2)(2016) 95107.Joao Vitor Vieira de Siqueira, Lucas Garrido Almeida, Otavio Bruno, Zica,Ingred Batista Brum, Alberto Barcelo, Arise Garcia de Siqueira Galil, Impact ofobesity on hospitalizations and mortality, due to COVID-19: a systematic review,Obes. Res. Clin. Pract. 14 (5) (2020) 398403.Thanusha Ananthakumar, Nicholas R. Jones, Lisa Hinton, Aveyard Paul, Clinicalencounters about obesity: systematic review of patients perspectives, Clin. Obes.10 (1) (2020), e12347.R. Felso, S. Lohner, K. Hollody, Erhardt, D. Molnar, Relationship between sleepduration and childhood obesity: systematic review including the potentialunderlying mechanisms, Nutr. Metabol. Cardiovasc. Dis. 27 (9) (2017) 751761.A. Barbara, Kitchenham. Systematic review in software engineering, in:Proceedings of the 2nd International Workshop on Evidential Assessment ofSoftware Technologies - EAST 12, ACM Press, New York, New York, USA, 2012,p. 1.Safaei Mahmood, Shahla Asadi, Maha Driss, Wadii Boulila, Alsaeedi Abdullah,Chizari Hassan, Rusli Abdullah, Safaei Mitra, A systematic literature review onoutlier detection inwireless sensor networks, Symmetry 12 (3) (2020) 328.Sima Taheri, Shahla Asadi, Mehrbakhsh Nilashi, Rabab Ali Abumalloh, M. Nawaf,A. Ghabban, Salma Yasmin Mohd Yusuf, Eko Supriyanto, Sarminah Samad,A literature review on beneficial role of vitamins and trace elements: evidencefrom published clinical studies, J. Trace Elem. Med. Biol. 67 (2021) 126789.Jula Amin, Elankovan Sundararajan, Zalinda Othman, Cloud computing servicecomposition: a systematic literature review, Expert Syst. Appl. 41 (8) (2014)38093824.Shahla Asadi, Ab Razak, Hussin Che, Halina Mohamed Dahlan, Organizationalresearch in the field of Green IT: a systematic literature review from 2007 to2016, Telematics Inf. 34 (7) (2017) 11911249.Richard T. Watson, Jane Webster, Analysing the past to prepare for the future:writing a literature review a roadmap for release 2.0, J. Decis. Syst. 29 (3) (2020)129147.Omprakash Kaiwartya, , Abdul Hanan Abdullah, Yue Cao, Ayman Altameem,Mukesh Prasad, Chin Teng Lin, Xiulei Liu, Guidelines for Performing SystematicLiterature Reviews in Software Engineering, Technical report, Keele Universityand Durham University Joint Report:United Kingdom., 2016.Shahla Asadi, Rusli Abdullah, Yusmadi Yah, Nazir Shah, Understandinginstitutional repository in higher learning institutions: a systematic literaturereview and directions for future research, IEEE Access 7 (2019) 3524235263.Srinivas Nidhra, Muralidhar Yanamadala, Wasif Afzal, Richard Torkar,Knowledge transfer challenges and mitigation strategies in global softwaredevelopment-A systematic literature review and industrial validation, Int. J. Inf.Manag. 33 (2) (2013) 333355.Adela Hruby, Frank B. Hu, The Epidemiology of Obesity: A Big Picture 33, 2015,pp. 673689, 7.M. Safaei et al.Computers in Biology and Medicine 136 (2021) 104754[28] Cheong Kim, Francis Joseph Costello, Kun Chang Lee, Li Yuan, Chenyao Li,Predicting factors affecting adolescent obesity using general bayesian networkand what-if analysis, Int. J. Environ. Res. Publ. Health 16 (23) (2019) 4684.[29] Akira Ishida, Yushuang Li, Osami Matsuda, Emiko Ishida, Factors affecting adultoverweight and obesity in urban China, Pertanika J. Soc. Sci. Human. 28 (1)(2020) 503513.[30] Helen Cheng, Adrian Furnham, Personality traits, education, physical exercise,and childhood neurological function as independent predictors of adult obesity,PloS One 8 (11) (2013), e79586.[31] Simeon-Pierre Choukem, Joel Noutakdie Tochie, Aurelie T. Sibetcheu, JobertRichie Nansseu, P. Julian, Hamilton-Shield, Overweight/obesity and associatedcardiovascular risk factors in sub-Saharan African children and adolescents: ascoping review, Int. J. Pediatr. Endocrinol. 2020 (1) (2020) 6.[32] Amelia Ma, Gomez-Llorente, Raquel Romero, Natalia Chueca, Ana MartinezCanavate, Carolina Gomez-Llorente, Obesity and asthma: a missing link, Int. J.Mol. Sci. 18 (7) (2017) 1490.[33] Balaji Meriga, Muni Swamy Ganjayi, Brahma Naidu Parim, Phytocompounds aspotential agents to treat obesity-cardiovascular ailments, Cardiovasc. Hematol.Agents Med. Chem. 15 (2) (2018) 104120.[34] Abdul Gafoor Abdul Mubarak, Reynu Rajan, Kirubakaran Malapan, Vimal KumarVasudeavan, Nik Ritza Kosai Nik Mahmood, Tikfu Gee, Haron Ahmad, Patientand Procedure Selection for Bariatric and Metabolic Surgery in Malaysia- theMalaysian Consensus. Technical Report, 2021, 2.[35] Frank Qian, Matti A. Rookus, Goska Leslie, Harvey A. Risch, Mark H. Greene,M. Cora, Aalfs, A Adank Muriel, Julian Adlard, Bjarni A. Agnarsson,Munaza Ahmed, et al., Mendelian randomisation study of height and body massindex as modifiers of ovarian cancer risk in 22,588 brca1 and brca2 mutationcarriers, Br. J. Canc. 121 (2) (2019) 180192.[36] Sartorius Benn, Lennert J. Veerman, Mercy Manyema, Lumbwe Chola, and KarenHofman. Determinants of obesity and associated population attributability, SouthAfrica: empirical evidence from a national panel survey, 2008-2012, PloS One 10(6) (2015), e0130218.[37] Y. Sun, S. Wang, X. Sun, Estimating neighbourhood-level prevalence of adultobesity by socio-economic, behavioural and built environment factors in NewYork City, Publ. Health 186 (2020) 5762.[38] Helen Cheng, Montgomery Scott, Andy Green, Adrian Furnham, Biomedical,psychological, environmental and behavioural factors associated with adultobesity in a nationally representative sample, J. Publ. Health 42 (3) (2020)570578.[39] Abbasi Ali, Linda M. Peelen, Eva Corpeleijn, T. Yvonne, Van Der Schouw, RonaldP. Stolk, M. Annemieke, W. Spijkerman, L. Daphne, A. Van Der, G. Karel,M. Moons, Gerjan Navis, Stephan J.L. Bakker, Joline, W.J. Beulens, Predictionmodels for risk of developing type 2 diabetes: systematic literature search andindependent external validation study, BMJ 345 (7875) (2012) e5900e5900.[40] Quan Zou, Kaiyang Qu, Yamei Luo, Dehui Yin, Ying Ju, Hua Tang, Predictingdiabetes mellitus with machine learning techniques, Front. Genet. 9 (2018) 515.[41] Ziad Obermeyer, J. Ezekiel, Emanuel. Predicting the future - big data, machinelearning, and clinical medicine, N. Engl. J. Med. 375 (13) (2016) 12161219.[42] Balbir Singh, Hissam Tawfik, A machine learning approach for predicting weightgain risks in young adults, in: Conference Proceedings of 2019 10th InternationalConference on Dependable Systems, Services and Technologies, DESSERT, vol.2019, 2019, pp. 231234.[43] Animesh Acharjee, Zsuzsanna Ament, James A. West, Elizabeth Stanley, JulianL. Griffin, Integration of metabolomics, lipidomics and clinical data using amachine learning method, BMC Bioinf. 17 (S15) (2016) 440.[44] Tamara M. Dugan, S. Mukhopadhyay, A. Carroll, S. Downs, Machine learningtechniques for prediction of early childhood obesity, Appl. Clin. Inf. 6 (3) (2015)506520.[45] Katherine Ellis, Jacqueline Kerr, Suneeta Godbole, John Staudenmayer,Gert Lanckriet, Hip and wrist accelerometer algorithms for free-living behaviorclassification, Med. Sci. Sports Exerc. 48 (5) (2016) 933940.[46] Andreas Triantafyllidis, Eleftheria Polychronidou, Anastasios Alexiadis, CleiltonLima Rocha, Nogueira Douglas, Oliveira, S. Amanda, da Silva, Ananda LimaFreire, Crislanio Macedo, Igor Farias Sousa, Eriko Werbet, Elena Arredondo Lillo,Henar Gonzalez Luengo, Macarena Torrego Ellacura, Konstantinos Votis, andDimitrios Tzovaras. Computerized decision support and machine learningapplications for the prevention and treatment of childhood obesity: a systematicreview of the literature, Artif. Intell. Med. 104 (2020), 101844.[47] S. Arielle, Selya and Drake Anshutz. Machine learning for the classification ofobesity from dietary and physical activity patterns, in: Smart Innovation, Systemsand Technologies, vol. 93, Springer Science and Business Media DeutschlandGmbH, 2018, pp. 7797.[48] Muhammed Krsad Uar, Zeliha Uar, Fatih Koksal, Nihat Daldal, Estimation ofbody fat percentage using hybrid machine learning algorithms, Measurement 167(2021) 108173.[49] Syed Afroz Keramat, Khorshed Alam, Jeff Gow, J. Stuart, H. Biddle, Impact ofdisadvantaged neighborhoods and lifestyle factors on adult obesity: evidencefrom a 5-year cohort study in Australia, Am. J. Health Promot. 35 (1) (2021)2837.[50] M.Pinto Snehal, Pereira, L. Bianca, De Stavola, Nina T. Rogers, Rebecca Hardy,Rachel Cooper, Chris Power, Adult obesity and mid-life physical functioning intwo British birth cohorts: investigating the mediating role of physical inactivity,Int. J. Epidemiol. 49 (3) (2021) 845856.[51] Shaojing Sun, Jinbo He, Bin Shen, Xitao Fan, Yibei Chen, Xiaohui Yang, Obesityas a self-regulated epidemic: coverage of obesity in Chinese newspapers, Eat.Weight Disord. 26 (2) (2020) 569584.[52] Rajaa Al-Raddadi, Suhad M. Bahijri, Hanan A. Jambi, Ferns Gordon,Jaakko Tuomilehto, The prevalence of obesity and overweight, associateddemographic and lifestyle factors, and health status in the adult population ofJeddah, Saudi Arabia, Therapeut. Adv. Chron. Dis. 10 (2019),204062231987899.[53] Orien L. Tulp, Olayide F. Obidi, Temitope C. Oyesile, George P. Einstein, Theprevalence of adult obesity in Africa: a meta-analysis, Gene Rep. 11 (2018)124126.[54] Lecube Albert, Enric Sanchez, Susana Monereo, Gema Medina-Gomez,Diego Bellido, Manuel Jose, Garcia-Almeida, Purificacion Martinez De Icaya,Maria Mar Malagon, Goday Albert, Francisco Jose Tinahones, Factors accountingfor obesity and its perception among the adult Spanish population: data from1,000 computer-assisted telephone interviews, Obesity Facts 13 (4) (2020)322332.[55] Sara Nikookar, Mohammad Hassan Eftekhari, Hamidreza Tabatabaei,Maryam Ranjbarzahedani, Seyed Jalil Masoumi, Clinical Nutrition, FoodSciences, Medical Sciences, Health Sciences, Medical Sciences, and Article Info.Overweight, Obesity, and its Associated Factors in Adult Women Referring toHealth Centers in Shiraz. Technical Report, 2017, 1.[56] Claire Yang, Yang, Moira P. Johnson, Kristen M. Schorpp, Courtney E. Boen,Kathleen Mullan Harris, Young adult risk factors for cancer: obesity,inflammation, and sociobehavioral mechanisms, Am. J. Prev. Med. 53 (3) (2017)S21S29.[57] Renata Micha, Shahab Khatibzadeh, Peilin Shi, Kathryn G. Andrews, RebeccaE. Engell, Dariush Mozaffarian, Global, regional and national consumption ofmajor food groups in 1990 and 2010: a systematic analysis including 266country-specific nutrition surveys worldwide on behalf of the Global Burden ofDiseases Nutrition and Chronic Diseases, Expert Group (Nutri. Open 5 (9) (2015)8705.[58] Annibale Cois, Candy Day, Obesity trends and risk factors in the South Africanadult population, BMC Obesity 2 (1) (2015) 110.[59] Haiyuan Shi, Bo Jiang, Joshua Dao, Wei Sim, Zhi Zhen Chum, Noreffendy Bin Ali,Mun Heng Toh, Factors associated with obesity: a case-control study of youngadult Singaporean males, Mil. Med. 179 (10) (2014) 11581165.[60] Yeow Nyin Ang, Bee Suan Wee, Bee Koon Poh, Mohd Noor Ismail, Multifactorialinfluences of childhood obesity, Curr. Obes. Rep. 2 (1) (2013) 1022.[61] Josefina Bressan, Fernanda de Carvalho Vidigal, M. Helen Hermana, Hermsdorff.Social components of the obesity epidemic, Curr. Obes. Rep. 2 (1) (2013) 3241.[62] Lingren Todd, Vidhu Thaker, Cassandra Brady, Bahram Namjou,Stephanie Kennebeck, Jonathan Bickel, Patibandla Nandan, Yizhao Ni, L. Sara,Van Driest, Lixin Chen, Ashton Roach, Beth Cobb, Jacqueline Kirby, Josh Denny,Lisa Bailey-Davis, Marc S. Williams, Marsolo Keith, Imre Solti, Ingrid A. Holm,John Harley, Isaac S. Kohane, Guergana Savova, Nancy Crimmins, Developing analgorithm to detect early childhood obesity in two tertiary pediatric medicalcenters, Appl. Clin. Inf. 7 (3) (2016) 693706.[63] Tania Fernandez-Navarro, Irene Daz, Isabel Gutierrez-Daz, Javier RodrguezCarrio, Ana Suarez, G. Clara, De los Reyes-Gavilan, Miguel Gueimonde, NuriaSalazar, and Sonia Gonzalez. Exploring the interactions between serum free fattyacids and fecal microbiota in obesity through a machine learning algorithm, FoodRes. Int. 121 (2019) 533541.[64] N. Lazzarini, J. Runhaar, A.C. Bay-Jensen, C.S. Thudium, S.M.A. Bierma-Zeinstra,Y. Henrotin, J. Bacardit, A machine learning approach for the identification ofnew biomarkers for knee osteoarthritis development in overweight and obesewomen, Osteoarthritis Cartilage 25 (12) (2017) 20142021.[65] Parisa Pouladzadeh, Pallavi Kuhad, Sri Vijay Bharat Peddi, Abdulsalam Yassine,Shervin Shirmohammadi, Food calorie measurement using deep learning neuralnetwork, in: 2016 IEEE International Instrumentation and MeasurementTechnology Conference Proceedings, vol. 2016, IEEE, 2016, pp. 16. July.[66] Bassam Farran, Rihab AlWotayan, Hessa Alkandari, Dalia Al-Abdulrazzaq,Arshad Channanath, Thangavel Alphonse Thanaraj, Use of non-invasiveparameters and machine-learning algorithms for predicting future risk of type 2diabetes: a retrospective cohort study of health data from Kuwait, Front.Endocrinol. 10 (2019) 624.[67] Kapil Jindal, Niyati Baliyan, Prashant Singh Rana, Obesity prediction usingensemble machine learning approaches, in: Advances in Intelligent Systems andComputing, vol. 708, Springer Verlag, 2018, pp. 355362.[68] Jocelyn Dunstan, Marcela Aguirre, Magdalena Bastas, Claudia Nau, ThomasA. Glass, Felipe Tobar, Predicting nationwide obesity from food sales usingmachine learning, Health Inf. J. 26 (1) (2020) 652663.[69] Anar Taghiyev, Adem Alpaslan Altun, Sona Caglar, A Hybrid Approach based onMachine Learning to Identify the Causes of Obesity. Technical Report 2, 2020.[70] Isaac Machorro-Cano, Giner Alor-Hernandez, Mario Andres Paredes-Valverde,Uriel Ramos-Deonati, Jose Luis Sanchez-Cervantes, Lisbeth Rodrguez-Mazahua,PISIoT: a machine learning and IoT-based smart health platform for overweightand obesity control, Appl. Sci. 9 (15) (2019) 3037.[71] Jiao Wang, Zhiqiang Deng, Modeling and prediction of oyster norovirusoutbreaks along Gulf of Mexico Coast, Environ. Health Perspect. 124 (5) (2016)627633.[72] Bahaa Khalil, Adamowski Jan, Alaa El-Din Abdin, Eizeldin Mohamed, Estimationof water quality characteristics at ungauged sites using multiple linear regressionand canonical correlation analysis, in: American Society of Agricultural andBiological Engineers Annual International Meeting 2014, ASABE 2014 1, 2014,pp. 322331, 34.[73] C. Shu, T.B.M.J. Ouarda, Flood frequency analysis at ungauged sites usingartificial neural networks in canonical correlation analysis physiographic space,Water Resour. Res. 43 (7) (2007).15M. Safaei et al.Computers in Biology and Medicine 136 (2021) 104754[74] Ayan Chatterjee, Martin W. Gerdes, Santiago G. Martinez, Identification of riskfactors associated with obesity and overweight-a machine learning overview,Sensors 20 (9) (2020) 2734.[75] Tim L. Emmerzaal, Amanda J. Kiliaan, Deborah R. Gustafson, 2003-2013: adecade of body mass index, Alzheimers disease, and dementia, J. Alzheim. Dis.43 (3) (2015) 739755.[76] G. Cheng, C. Huang, H. Deng, H. Wang, Diabetes as a risk factor for dementia andmild cognitive impairment: a meta-analysis of longitudinal studies, Intern. Med.J. 42 (5) (2012) 484491.[77] Julie Hugo, Mary Ganguli, Dementia and cognitive impairment. Epidemiology,diagnosis, and treatment, Clin. Geriatr. Med. 30 (3) (2014) 421442.[78] Janana Niero Mazon, Aline Haas de Mello, Gabriela Kozuchovski Ferreira,Gislaine Tezza Rezin, The impact of obesity on neurodegenerative diseases, LifeSci. 182 (2017) 2228.[79] Louis A. Profenno, Anton P. Porsteinsson, Stephen V. Faraone, Meta-analysis ofAlzheimers disease risk with obesity, diabetes, and related disorders, Biol.Psychiatr. 67 (6) (2010) 505512.[80] Taianah Almeida Barroso, Lucas Braga Marins, Renata Alves, Ana Caroline SouzaGonalves, Sergio Girao Barroso, Gabrielle de Souza Rocha, Association of centralobesity with the incidence of cardiovascular diseases and risk factors, Int. J.Cardiovasc. Sci. 30 (5) (2017) 416424.[81] Andrew Elagizi, Sergey Kachur, Carl J. Lavie, Salvatore Carbone,Ambarish Pandey, Francisco B. Ortega, Richard V. Milani, An overview andupdate on obesity and the obesity paradox in cardiovascular diseases, Prog.Cardiovasc. Dis. 61 (2) (2018) 142150.[82] Carl J. Lavie, Alban De Schutter, Parham Parto, Eiman Jahangir, Peter Kokkinos,Francisco B. Ortega, Arena Ross, Richard V. Milani, Obesity and prevalence ofcardiovascular diseases and prognosis-the obesity paradox updated, Prog.Cardiovasc. Dis. 58 (5) (2016) 537547.[83] Richard K. Lee, Doreen Chung, Bilal Chughtai, Alexis E. Te, Steven A. Kaplan,Central obesity as measured by waist circumference is predictive of severity oflower urinary tract symptoms, BJU Int. 110 (4) (2012) 540545.[84] Dyandra Parikesit, Chaidir Arief Mochtar, Rainy Umbas, Agus Rizal ArdyHariandy Hamid, The impact of obesity towards prostate diseases, Prostate Int. 4(1) (2016) 16.[85] Melissa Xanthopoulos, Ignacio E. Tapia, Obesity and common respiratory diseasesin children, Paediatr. Respir. Rev. 23 (2017) 6871.[86] Mathilde Versini, Pierre Yves Jeandel, Eric Rosenthal, Yehuda Shoenfeld, Obesityin autoimmune diseases: not a passive bystander, in: Mosaic of Autoimmunity:the Novel Factors of Autoimmune Diseases, Elsevier, 2019, pp. 343372.[87] Ataey Amin, Elnaz Jafarvand, Davoud Adham, Eslam Moradi-Asl, Therelationship between obesity, overweight, and the human development index inworld health organization eastern mediterranean region countries, J. Prev. Med.Public Health 53 (2) (2020) 98105.[88] Fanny Kilpi, Laura Webber, Abdulrahman Musaigner, Amina Aitsi-Selmi,Tim Marsh, Ketevan Rtveladze, Klim McPherson, Martin Brown, Alarmingpredictions for obesity and non-communicable diseases in the Middle East, Publ.Health Nutr. 17 (5) (2014) 10781086.[89] P. Fergus, A. Hussain, J. Hearty, S. Fairclough, L. Boddy, K.A. Mackintosh,G. Stratton, N.D. Ridgers, Naeem Radi, A machine learning approach to measureand monitor physical activity in children to help fight overweight and obesity, in:Lecture Notes in Computer Science (Including Subseries Lecture Notes inArtificial Intelligence and Lecture Notes in Bioinformatics), vol. 9226, SpringerVerlag, 2015, pp. 676688.[90] James D. Pleuss, Kevin Talty, Steven Morse, Patrick Kuiper, Michael Scioletti,Steven B. Heymsfield, Diana M. Thomas, A machine learning approach relating3D body scans to body composition in humans, Eur. J. Clin. Nutr. 73 (2) (2019)200208.[91] Mehak Gupta, Thao-Ly Phan, Timothy Bunnell, Rahmatollah Beheshti, ObesityPrediction with EHR Data: A Deep Learning Approach with InterpretableElements, arXiv preprint arXiv:1912.02655, page arXiv: 1912.02655, 2019.[92] Lucas de Moura Carvalho, Vasco Furtado, Jose Eurico de Vasconcelos Filho,Carminda Maria Goersch Fontenele Lamboglia, Using machine learning forevaluating the quality of exercises in a mobile exergame for tackling obesity inchildren, in: Lecture Notes in Networks and Systems, vol. 16, Springer, 2018,pp. 373390.[93] Mathias J. Gerl, Christian Klose, Michal A. Surma, Celine Fernandez,Olle Melander, Satu Mannisto, Katja Borodulin, Aki S. Havulinna,Veikko Salomaa, Elina Ikonen, Carlo V. Cannistraci, Kai Simons, Machinelearning of human plasma lipidomes for obesity estimation in a large populationcohort, PLoS Biol. 17 (10) (2019), e3000443.[94] Casimiro Aday Curbelo Montanez, Fergus Paul, Abir Hussain, Dhiya Al-Jumeily,Basma Abdulaimma, Jade Hind, Naeem Radi, Machine learning approaches forthe prediction of obesity using publicly available genetic profiles, in: Proceedingsof the International Joint Conference on Neural Networks, vol. 2017, 2017,pp. 27432750. May.[95] Wiechmann Paul, Karina Lora, Branscum Paul, Jicheng Fu, Identifyingdiscriminative attributes to gain insights regarding child obesity in hispanicpreschoolers using machine learning techniques, in: Proceedings - InternationalConference on Tools with Artificial Intelligence, ICTAI, 2018, pp. 1115.[96] Xueqin Pang, Christopher B. Forrest, Felice Le-Scherban, J. Aaron, Masino,Understanding early childhood obesity via interpretation of machine learningmodel predictions, in: Proceedings - 18th IEEE International Conference onMachine Learning and Applications, vol. 2019, ICMLA, 2019, pp. 14381443.[97] Naomi Christianne Pereira, Jessica DSouza, Parth Rana, Supriya Solaskar,Obesity related disease prediction from healthcare communities using machine[98][99][100][101][102][103][104][105][106][107][108][109][110][111][112][113][114][115][116][117][118][119][120][121][122]16learning, in: 2019 10th International Conference on Computing, Communicationand Networking Technologies, vol. 2019, ICCCNT, 2019, pp. 17.Kunal Rajput, Girija Chetty, Rachel Davey, Obesity and Co-morbidity detection inclinical text using deep learning and machine learning techniques, in:Proceedings - 2018 5th Asia-Pacific World Congress on Computer Science andEngineering, APWC on CSE, vol. 2018, IEEE, 2018, pp. 5156.Milla Kibble, A. Suleiman, Khan, Muhammad Ammad-Ud-Din,Sailalitha Bollepalli, Teemu Palviainen, Jaakko Kaprio, Kirsi H. Pietilainen,Miina Ollikainen, An Integrative Machine Learning Approach to DiscoveringMulti-Level Molecular Mechanisms of Obesity Using Data from MonozygoticTwin Pairs: Machine Learning Applied to Obesity, vol. 7, Royal Society OpenScience, 2020, p. 200872, 10.David Scheinker, Areli Valencia, Fatima Rodriguez, Identification of factorsassociated with variation in US county-level obesity prevalence rates usingepidemiologic vs machine learning models, JAMA Network open 2 (4) (2019),e192884.Zeyu Zheng, Karen Ruggiero, Using machine learning to predict obesity in highschool students, in: Proceedings - 2017 IEEE International Conference onBioinformatics and Biomedicine, BIBM, vol. 2017, 2017, pp. 21322138.Hsin Yao Wang, Shih Cheng Chang, Wan Ying Lin, Chun Hsien Chen, SzuHsien Chiang, Kai Yao Huang, Bo Yu Chu, Jih Jang, Lu, Tzong Yi Lee, Machinelearning-based method for obesity risk evaluation using single-nucleotidepolymorphisms derived from next-generation sequencing, J. Comput. Biol. 25(12) (2018) 13471360.Mengdi Xia, Kaixiang Liu, Jie Feng, Zaiqiong Zheng, Xisheng Xie, Prevalence andrisk factors of type 2 diabetes and prediabetes among 53,288 middle-aged andelderly adults in China: a cross-sectional study, Diabetes, Metab. Syndrome Obes.Targets Ther. 14 (19751985) 2021.Michele Lessa de Oliveira, Leonor Maria Pacheco Santos, Everton Nunes da Silva,Direct healthcare cost of obesity in Brazil: an application of the cost-of-illnessmethod from the perspective of the public health system in 2011, PloS One 10 (4)(2015), e0121160.Tabares2017health. Health effects of overweight and obesity in 195 countriesover 25 years, N. Engl. J. Med. 377 (1) (2017) 1327.M. Lette, W.J.E. Bemelmans, J. Breda, L.C.J. Slobbe, J. Dias, H.C. Boshuizen,Health care costs attributable to overweight calculated in a standardized way forthree European countries, Eur. J. Health Econ. 17 (1) (2016) 6169.Katie Power, M.M. Davies, R. Hargest, S. Phillips, J. Torkington, C. Morris, A casecontrol study of risk factors for wound infection in a colorectal unit, Ann. R. Coll.Surg. Engl. 96 (1) (2014) 3740.R. Huttunen, J. Syrjanen, Obesity and the risk and outcome of infection, Int. J.Obes. 37 (3) (2013) 333340.A. Atamna, A. Elis, E. Gilady, L. Gitter-Azulay, J. Bishara, How obesity impactsoutcomes of infectious diseases, Eur. J. Clin. Microbiol. Infect. Dis. 36 (3) (2017)585591.Matthias Blher, Clinical relevance of adipokines, Diabetes Metabol. J. 36 (5)(2012) 317327.William H. Dietz, Loel S. Solomon, Nico Pronk, Sarah K. Ziegenhorn,Marion Standish, Matt M. Longjohn, An integrated framework for the preventionand treatment of obesity and its related chronic diseases, Health Aff. 34 (9)(2015) 14561463.Flora Ippoliti, Nicoletta Canitano, Rita Businaro, Stress and Obesity as RiskFactors in Cardiovascular Diseases: A Neuroimmune Perspective 8, 2013,pp. 212226, 1.Sren Jepsen, Suvan Jean, Deschner James, The association of periodontaldiseases with metabolic syndrome and obesity, Periodontol. 2000 83 (1) (2020)125153.Wolfgang Kopp, How western diet and lifestyle drive the pandemic of obesity andcivilization diseases, Diabetes, Metab. Syndrome Obes. Targets Ther. 12 (2019)22212236.C.J. Lavie, R.V. Milani, H.O. Ventura, Obesity and the obesity paradox incardiovascular diseases, Clin. Pharmacol. Therapeut. 90 (1) (2011) 2325.Carl J. Lavie, Martin A. Alpert, Arena Ross, Mandeep R. Mehra, Richard V. Milani,Hector O. Ventura, Impact of Obesity and the Obesity Paradox on Prevalence andPrognosis in Heart Failure, 2013.Carl J. Lavie, Arena Ross, Martin A. Alpert, Richard V. Milani, Hector O. Ventura,Management of cardiovascular diseases in patients with obesity, Nat. Rev.Cardiol. 15 (1) (2018) 4556.Demetrios Petrakis, Loukia Vassilopoulou, Charalampos Mamoulakis,Christos Psycharakis, Aliki Anifantaki, Stavros Sifakis, Anca Docea,John Tsiaoussis, Antonios Makrigiannakis, Aristides Tsatsakis, Endocrinedisruptors leading to obesity and related diseases, Int. J. Environ. Res. Publ.Health 14 (10) (2017) 1282.Marie-Eve Piche, Andre Tchernof, Jean-Pierre Despres, Obesity phenotypes,diabetes, and cardiovascular diseases, Circ. Res. 126 (11) (2020) 14771500.Alessandra Vecchie, Dallegri Franco, Federico Carbone, Aldo Bonaventura,Luca Liberale, Piero Portincasa, Gema Frhbeck, Fabrizio Montecucco, Obesityphenotypes and their paradoxical association with cardiovascular diseases, Eur. J.Intern. Med. 48 (617) (2018).Yang Zhou, Ning Zhang, The burden of overweight and obesity on long-term careand medicaid financing, Med. Care 52 (7) (2014) 658663.David A. Alter, Harindra C. Wijeysundera, Barry Franklin, Peter C. Austin,Alice Chong, Paul I. Oh, Jack V. Tu, Therese A. Stukel, Obesity, lifestyle riskfactors, and health service outcomes among healthy middle-aged adults inCanada, BMC Health Serv. Res. 12 (1) (2012) 238.M. Safaei et al.Computers in Biology and Medicine 136 (2021) 104754[123] S.K. Garg, H. Maurer, K. Reed, R. Selagamsetty, Diabetes and cancer: two diseaseswith obesity as a common risk factor, Diabetes Obes. Metabol. 16 (2) (2014)97110.[124] Paiboon Pitayatienanan, Rukmanee Butchon, Jomkwan Yothasamut,Wichai Aekplakorn, Teerawattananon Yot, Naeti Suksomboon,Montarat Thavorncharoensap, Economic costs of obesity in Thailand: aretrospective cost-of-illness study, BMC Health Serv. Res. 14 (1) (2014) 146.[125] Philipp E. Scherer, Joseph A. Hill, Obesity, diabetes, and cardiovascular diseases,Circ. Res. 118 (11) (2016) 17031705.[126] Shalini Verma, M. Ejaz Hussain, Obesity and diabetes: an update, DiabetesMetabol. Syndr.: Clin. Res. Rev. 11 (1) (2017) 7379.[127] Pouria Khosravi, Cameron Newton, Azadeh Rezvani, Management innovation: asystematic review and meta-analysis of past decades of research, Eur. Manag. J.37 (6) (2019) 694707.[128] Cynthia A. Martin-Jimenez, Angela Garca-Vega, Ricardo Cabezas,Gjumrakch Aliev, Valentina Echeverria, Janneth Gonzalez, E. George, Barreto,Astrocytes and endoplasmic reticulum stress: a bridge between obesity andneurodegenerative diseases, Prog. Neurobiol. 158 (2017) 4568.[129] M.A. Sede, A.O. Ehizele, Relationship between obesity and oral diseases, Niger. J.Clin. Pract. 17 (6) (2014) 683690.[130] S.M. Chopra, A. Misra, S. Gulati, R. Gupta, Overweight, obesity and related noncommunicable diseases in Asian Indian girls and women, Eur. J. Clin. Nutr. 67 (7)(2013) 688696.[131] Y. Wang, L. Wang, W. Qu, New national data show alarming increase in obesityand noncommunicable chronic diseases in China, Eur. J. Clin. Nutr. 71 (1) (2017)149150.[132] Elisa Gremese, Barbara Tolusso, Maria Rita Gigante, Gianfranco Ferraccioli,Obesity as a risk and severity factor in rheumatic diseases (autoimmune chronicinflammatory diseases) 5 (11) (2014) 576.[133] Youn Su, Nam. Obesity-related digestive diseases and their pathophysiology, GutLiver 11 (3) (2017) 323334.[134] Christopher Zammit, Helen Liddicoat, Ian Moonsie, Himender Makker, Obesityand respiratory diseases, Am. J. Clin. Hypn. 53 (4) (2011) 335343.[135] S.W. Ng, S. Zaghloul, H.I. Ali, G. Harrison, B.M. Popkin, The prevalence andtrends of overweight, obesity and nutrition-related non-communicable diseases inthe Arabian Gulf States, Obes. Rev. 12 (1) (2011) 113.[136] Laura Webber, Fanny Kilpi, Tim Marsh, Ketevan Rtveladze, Martin Brown,Klim McPherson, High rates of obesity and non-communicable diseases predictedacross Latin America, PloS One 7 (8) (2012), e39589.[137] Edda Maria Capodaglio, Participatory ergonomics for the reduction ofmusculoskeletal exposure of maintenance workers, Int. J. Occup. Saf. Ergon. 111(2020).[138] Veronica Cimolin, Manuela Galli, Luca Vismara, Giorgio Albertini,Alessandro Sartorio, Paolo Capodaglio, Gait pattern in lean and obeseadolescents, Int. J. Rehabil. Res. 38 (1) (2015) 4048.[139] Cintia Renata Sousa-Gonalves, Gabriella Tringali, Sofia Tamini, Roberta DeMicheli, Davide Soranna, Redha Taiar, Danbia Sa-Caputo, Eloa MoreiraMarconi, Laisa Paineiras-Domingos, Mario Bernardo-Filho, Alessandro Sartorio,Acute effects of whole-body vibration alone or in combination with maximalvoluntary contractions on cardiorespiratory, musculoskeletal, and neuromotorfitness in obese male adolescents, Dose-Response 17 (4) (2019),155932581989049.[140] Nathalie Michels, Barbara Vanaelst, Vyncke Krishna, Isabelle Sioen,Inge Huybrechts, Tineke De Vriendt, Stefaan De Henauw, Childrens Bodycomposition and Stress - the ChiBS study: aims, design, methods, population andparticipation characteristics, Arch. Publ. Health 70 (1) (2012) 113.[141] K.W. DeGregory, P. Kuiper, T. DeSilvio, J.D. Pleuss, R. Miller, J.W. Roginski, C.B. Fisher, D. Harness, S. Viswanath, S.B. Heymsfield, et al., A review of machinelearning in obesity, Obes. Rev. 19 (5) (2018) 668685.17